Hey Flipper, which way will the wind blow for you
Post# of 30067
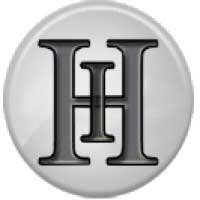
In statistical significance testing, the p-value is the probability of obtaining a test statistic result at least as extreme as the one that was actually observed, assuming that the null hypothesis is true.[1][2] A researcher will often "reject the null hypothesis" when the p-value turns out to be less than a predetermined significance level, often 0.05[3][4] or 0.01. Such a result indicates that the observed result would be highly unlikely under the null hypothesis. Many common statistical tests, such as chi-squared tests or Student's t-test, produce test statistics which can be interpreted using p-values.
In a statistical test, sample results are compared to possible population conditions by way of two competing hypotheses: the null hypothesis is a neutral or "uninteresting" statement about a population, such as "no change" in the value of a parameter from a previous known value or "no difference" between two groups; the other, the alternative (or research) hypothesis is the "interesting" statement that the person performing the test would like to conclude if the data will allow it. The p-value is the probability of obtaining the observed sample results (or a more extreme result) when the null hypothesis is actually true. If this p-value is very small, usually less than or equal to a threshold value previously chosen called the significance level (traditionally 5% or 1% [5]), it suggests that the observed data is inconsistent with the assumption that the null hypothesis is true, and thus that hypothesis must be rejected and the other hypothesis accepted as true.
An informal interpretation of a p-value, based on a significance level of about 10%, might be:
: very strong presumption against null hypothesis
: strong presumption against null hypothesis
: low presumption against null hypothesis
: no presumption against the null hypothesis
A new Bayesian inference approach highlights that these threshold values are too optimistic and explain the lack of reproducibility of scientific studies, suggesting a p < 0.001 or 0.0053.[6] However, a follow-up article illustrates that these more stringent threshold values are not absolute, but rather arise from "the discrepancy between p-values and Bayes factors", and are not a complete solution to the problem of reproducibility.[7]
The p-value is a key concept in the approach of Ronald Fisher, where he uses it to measure the weight of the data against a specified hypothesis, and as a guideline to ignore data that does not reach a specified significance level.[5] Fisher's approach does not involve any alternative hypothesis, which is instead a feature of the Neyman–Pearson approach. The p-value should not be confused with the significance level α in the Neyman–Pearson approach or the Type I error rate [false positive rate]. Fundamentally, the p-value does not in itself support reasoning about the probabilities of hypotheses, nor choosing between different hypotheses – it is simply a measure of how likely the data (or a more "extreme" version of it) were to have occurred, assuming the null hypothesis is true.[8]
Statistical hypothesis tests making use of p-values are commonly used in many fields of science and social sciences, such as economics, psychology,[9] biology, criminal justice and criminology, and sociology.[10]
Depending on which style guide is applied, the "p" is styled either italic or not, capitalized or not, and hyphenated or not (p-value, p value, P-value, P value, p-value, p value, P-value, P value).

