Innovative AIoT Framework Enhances Smart Home Functionality
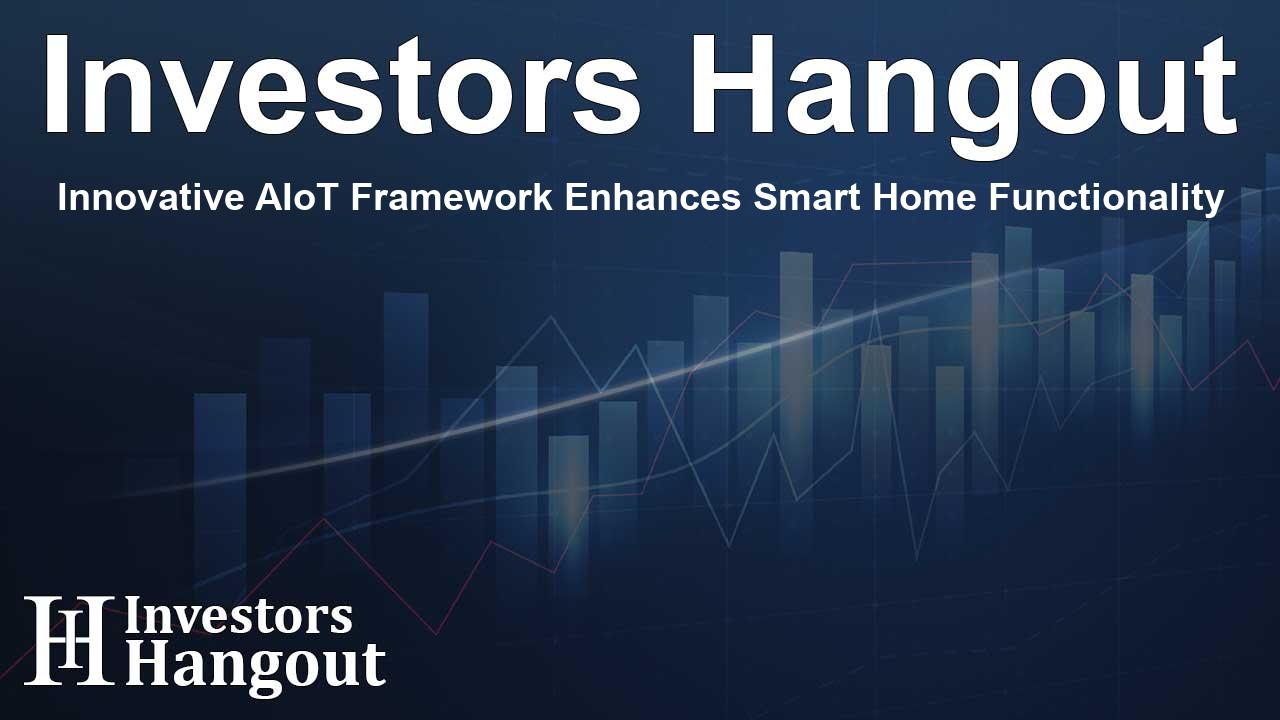
Enhancing Smart Home Security with AIoT Technologies
Artificial Intelligence of Things (AIoT) is gaining traction due to its broad range of applications. A team at Incheon National University has recently unveiled a cutting-edge AIoT framework known as MSF-Net. This innovative framework is designed to accurately recognize human activities through WiFi signals, thus paving the way for enhanced smart home security.
Understanding the Significance of AIoT
The essence of AIoT lies in its ability to harmonize Artificial Intelligence with the Internet of Things. Unlike traditional IoT systems that send data to external processors for analysis, AIoT enables devices to interpret data locally and in real-time. This allows for smart decision-making, making AIoT increasingly preferred in fields such as intelligent manufacturing, healthcare monitoring, and notably, smart home environments.
The Importance of Activity Recognition in Smart Homes
In the context of smart homes, the need for accurate human activity recognition is paramount. Such recognition facilitates the identification of various tasks like cooking or exercising, allowing the AIoT system to make adjustments accordingly. For instance, it can automatically modify lighting or change the music based on the identified activity, positively impacting user experience while also enhancing energy efficiency. Moreover, WiFi-based motion recognition shows great potential owing to the widespread availability of WiFi infrastructure, the respect for user privacy it offers, and its cost-effectiveness.
Details of the MSF-Net Framework
Recently, the research team led by Professor Gwanggil Jeon at Incheon National University developed the MSF-Net framework, focused on recognizing human activities through WiFi data. This research showcases a firm dedication to overcoming challenges typically faced in environment-sensitive scenarios. The team published their findings in the IEEE Internet of Things Journal.
Research Background and Goals
Professor Jeon elaborated on the impetus for this study stating, "WiFi-based human activity recognition is on the rise in smart home applications. However, challenges surrounding environmental factors can lead to performance instability. Our primary objective was to address these issues effectively." This research demonstrates the potential for robust AIoT applications in various sectors.
Structure and Functionality of MSF-Net
MSF-Net employs a sophisticated deep learning architecture that achieves both coarse and fine activity recognition utilizing Channel State Information (CSI). The framework consists of three key elements: a dual-stream structure utilizing short-time Fourier transform and discrete wavelet transform, a transformer for efficient feature extraction, and an attention-based fusion branch that enhances cross-model integration.
Performance Validation and Future Implications
The research team validated the framework's performance, achieving impressive Cohen's Kappa scores of 91.82%, 69.76%, 85.91%, and 75.66% on multiple datasets, which demonstrates its superiority over contemporary methodologies in the domain of WiFi-based activity recognition.
The implications of this study are profound. Professor Jeon concluded, "The multimodal frequency fusion technique significantly improves the accuracy of recognition, opening doors for practical applications in diverse areas ranging from smart homes to elderly care and rehabilitation medicine. This technology can potentially enhance quality of life by enabling non-intrusive health monitoring systems.”
Conclusion: The Future of AIoT in Smart Homes
Ultimately, the innovative application of WiFi technology to recognize human activities, as illustrated in this research, promises to enhance life's convenience and safety. The future is bright for AIoT technologies as they continue to evolve, making homes smarter and more efficient for everyone.
Frequently Asked Questions
What is the MSF-Net Framework?
MSF-Net is an AIoT framework developed at Incheon National University that uses WiFi signals to accurately recognize human activities, enhancing smart home security.
Who led the research behind MSF-Net?
The research was led by Professor Gwanggil Jeon from the College of Information Technology at Incheon National University.
How does the MSF-Net Framework work?
MSF-Net utilizes a combination of deep learning techniques and signal processing methods to refine human activity recognition accuracy by leveraging Channel State Information.
What applications can benefit from MSF-Net?
MSF-Net can be applied in various domains, including smart home environments, elderly care, rehabilitation, and non-intrusive health monitoring systems.
What are the expected outcomes of using MSF-Net in smart homes?
Using MSF-Net is expected to improve user experience by automating smart device adjustments based on recognized activities, thereby enhancing convenience and energy efficiency.
About The Author
Contact Hannah Lewis privately here. Or send an email with ATTN: Hannah Lewis as the subject to contact@investorshangout.com.
About Investors Hangout
Investors Hangout is a leading online stock forum for financial discussion and learning, offering a wide range of free tools and resources. It draws in traders of all levels, who exchange market knowledge, investigate trading tactics, and keep an eye on industry developments in real time. Featuring financial articles, stock message boards, quotes, charts, company profiles, and live news updates. Through cooperative learning and a wealth of informational resources, it helps users from novices creating their first portfolios to experts honing their techniques. Join Investors Hangout today: https://investorshangout.com/
The content of this article is based on factual, publicly available information and does not represent legal, financial, or investment advice. Investors Hangout does not offer financial advice, and the author is not a licensed financial advisor. Consult a qualified advisor before making any financial or investment decisions based on this article. This article should not be considered advice to purchase, sell, or hold any securities or other investments. If any of the material provided here is inaccurate, please contact us for corrections.