Unlocking the Future: MicroCloud Hologram's Quantum Breakthrough
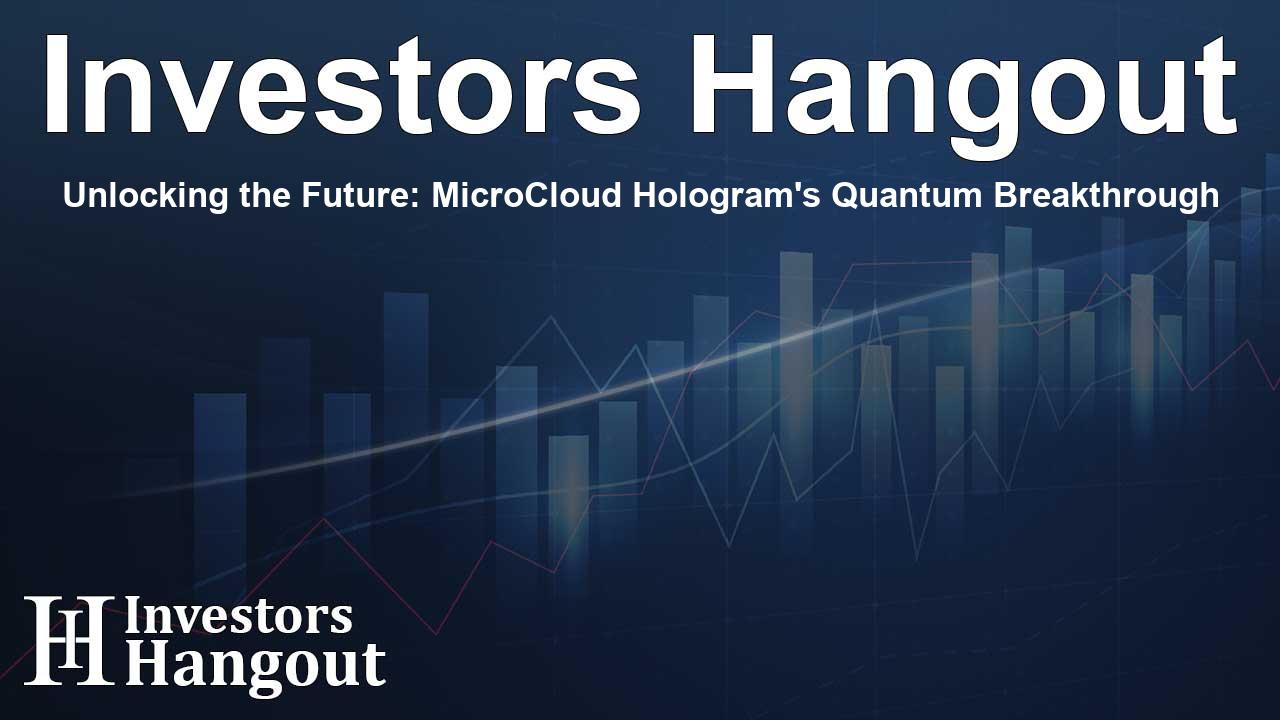
MicroCloud Hologram's Quantum Leap in AI Technology
MicroCloud Hologram Inc. (NASDAQ: HOLO), a progressive technology service provider, has developed groundbreaking quantum algorithm technology specifically designed for deep convolutional neural network (CNN) exchange submissions. This innovative approach aims to tackle the computational challenges that traditional CNNs face while harnessing the unique capabilities offered by quantum computing. Central to this advancement is the Quantum Convolutional Neural Network (QCNN), which not only mimics the output of classical CNNs but also addresses common quantum computing difficulties, particularly in executing nonlinear operations.
What Makes QCNN Stand Out?
The primary foundation of this technology is its ability to employ quantum circuits to successfully implement nonlinear activation functions and pooling operations within a quantum framework. This capability significantly enhances efficiency in both forward and backward propagation during the training of deep neural networks. Such enhancements provide critical support for complex tasks, positioning HOLO at the forefront of technological advancements.
Efficient Data Encoding
From a technical standpoint, the QCNN incorporates several key components that enhance its functionality. Initially, it features an input method that utilizes quantum state encoding. This technique enables high-dimensional data to be mapped into quantum states. By leveraging the properties of quantum superposition and entanglement, HOLO allows for parallel convolution operations in high-dimensional space, thereby streamlining computational processes.
Quantum Convolution Kernels
Additionally, HOLO engineered a series of quantum convolution kernels implemented through unitary operations. These kernels proficiently extract local features from input data, resulting in faster convolution achieved at quantum speed. In addressing the need for nonlinear activation functions, a measurement-based approach is utilized. This technique performs partial measurements on quantum states, achieving nonlinear mappings while preserving the essential properties of quantum superposition.
Advancements in Quantum Learning Techniques
The QCNN's design also includes efficient pooling operations that reduce feature dimensions through quantum state reduction measurements. In terms of training optimization, HOLO has introduced an innovative algorithm based on quantum gradient computations. Merging this with gradient descent methods results in agile updates of network parameters, which is crucial for improving model accuracy.
Real-World Applications
The QCNN technology is promising not just theoretically, but it holds considerable applicability in the real world. In fields like image recognition, its performance enhancements allow for rapid and accurate analysis of medical images, facilitating timely diagnostics. Furthermore, the capabilities of QCNN can enhance real-time processing in autonomous driving, thereby boosting safety and reliability. The potential extends to natural language processing and financial data analysis, showcasing the versatile applications of this technology.
Challenges Ahead for Quantum Innovations
Despite the remarkable strides made with QCNN, challenges remain evident for future research. Optimizing quantum circuits for larger datasets and more complex tasks is an avenue in need of exploration. Additionally, hardware limitations—such as noise interference and qubit constraints—pose significant obstacles to progression. Addressing these difficulties requires continual innovation in quantum algorithm design alongside a keen observation of developments within quantum hardware.
The Future of Quantum Convolutional Neural Networks
As an innovative framework within the deep learning landscape, QCNN presents new avenues for the practical application of quantum computing, resulting in exciting possibilities for the future of artificial intelligence. HOLO's advancements signal not just a breakthrough in quantum algorithm technology for CNN exchange submissions but also an essential milestone in intelligent computing’s evolution.
Looking forward, the evolving nature of quantum convolutional networks unveils substantial potential as advancements in quantum computing continue. The significance of these technologies extends beyond current computational limitations, introducing fresh perspectives within deep learning. The capabilities inherent in quantum computing—such as parallelism and superposition—allow QCNN to process high-dimensional data efficiently, indicating robust adaptability to complex environments.
Thus, by synergizing with industry needs, QCNN appears poised to play an indispensable role across sectors such as healthcare, transportation, and finance, contributing significantly to technological and socioeconomic progress.
About MicroCloud Hologram Inc.
MicroCloud is steadfast in offering state-of-the-art holographic technology services globally. Their offerings encompass high-precision holographic light detection and ranging (LiDAR) solutions, exclusive architecture design for holographic LiDAR point cloud algorithms, and advanced technical holographic imaging solutions. MicroCloud's expert team is engaged in designing holographic sensor chips and innovative vehicle intelligence technologies, aimed at ensuring dependable advanced driver assistance systems (ADAS).
Moreover, their holographic digital twin technology services present exceptional capabilities to capture shapes and objects in 3D holographic form via a unique resource library comprising software, spatial data, and advanced capture techniques.
Frequently Asked Questions
What is MicroCloud Hologram Inc. known for?
MicroCloud Hologram Inc. is recognized for its innovative holographic technology services, including advanced LiDAR solutions and quantum computing advancements.
How does the QCNN technology improve traditional CNNs?
QCNN enhances traditional CNNs by addressing computational bottlenecks through quantum computing capabilities, allowing for efficient nonlinear operations and reduced complexity.
What are the potential applications of QCNN?
QCNN can be applied in various fields, including healthcare for medical imaging, autonomous driving for real-time data processing, and in finance for data analysis.
What challenges does QCNN face moving forward?
Future challenges for QCNN include optimizing it for larger datasets, overcoming hardware limitations, and addressing potential noise interference in computations.
What does the future hold for quantum computing and AI?
The synergy of quantum computing and AI holds significant promise, presenting new opportunities for intelligent systems across various industries.
About The Author
Contact Dylan Bailey privately here. Or send an email with ATTN: Dylan Bailey as the subject to contact@investorshangout.com.
About Investors Hangout
Investors Hangout is a leading online stock forum for financial discussion and learning, offering a wide range of free tools and resources. It draws in traders of all levels, who exchange market knowledge, investigate trading tactics, and keep an eye on industry developments in real time. Featuring financial articles, stock message boards, quotes, charts, company profiles, and live news updates. Through cooperative learning and a wealth of informational resources, it helps users from novices creating their first portfolios to experts honing their techniques. Join Investors Hangout today: https://investorshangout.com/
The content of this article is based on factual, publicly available information and does not represent legal, financial, or investment advice. Investors Hangout does not offer financial advice, and the author is not a licensed financial advisor. Consult a qualified advisor before making any financial or investment decisions based on this article. This article should not be considered advice to purchase, sell, or hold any securities or other investments. If any of the material provided here is inaccurate, please contact us for corrections.