Revolutionary Predictive Model Enhances Magnesium Alloy Safety
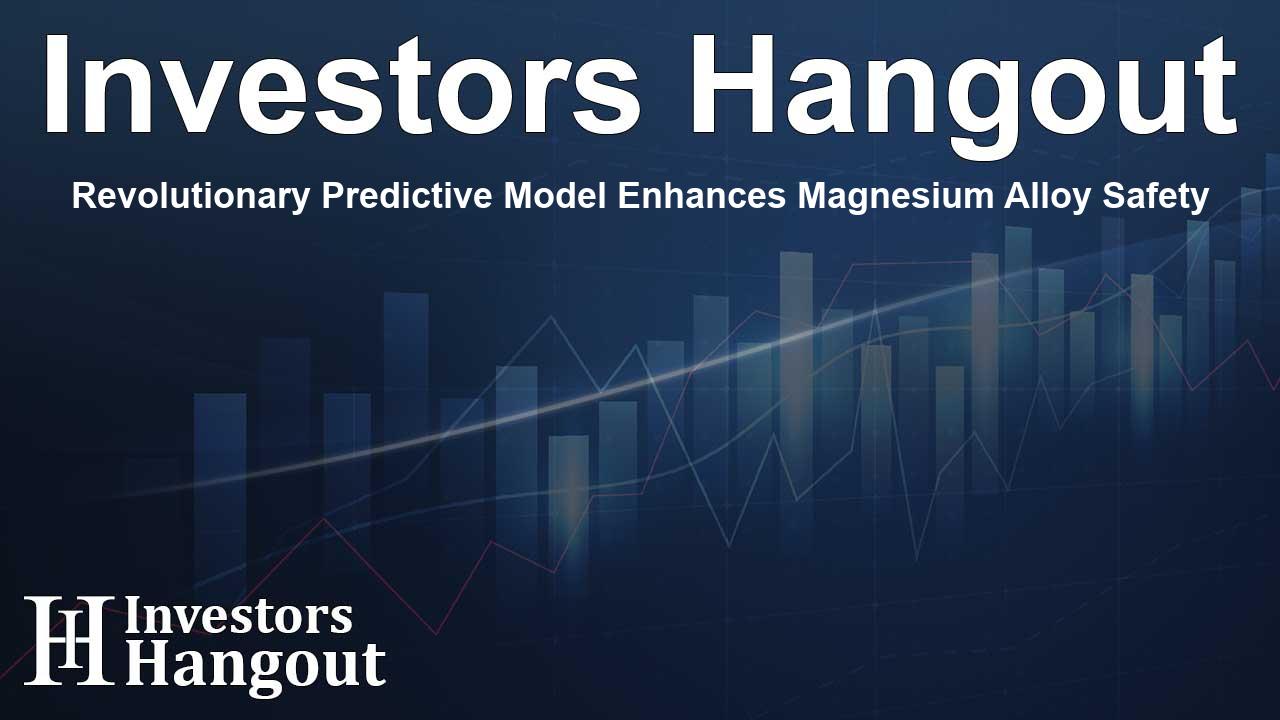
Groundbreaking Hybrid Model for Predicting Metal Wear
In a momentous development, researchers from Pusan National University have created a hybrid model that marries machine learning with traditional physical principles. This innovative approach aims to enhance the prediction of the fatigue life of magnesium alloys, which are increasingly essential in the automotive and aerospace sectors. Due to their unique properties, magnesium alloys are favored for lightweight designs, but predicting their durability under stress has remained a significant challenge.
The Challenge of Predicting Fatigue Life
Magnesium alloys are prized for their strength, lightweight nature, and manufacturability, making them ideal for vehicles and aircraft. Lighter designs allow for energy savings and lower emissions, which is crucial in today's eco-conscious environment. Nonetheless, the way magnesium alloys respond to stress is complex. Over time, these materials can encounter tiny cracks from the cyclic stresses they endure, posing risks in critical applications.
Historically, predicting when these cracks will emerge has proven difficult due to reliance on empirical models, which typically require constant recalibration to adapt to varying loading conditions. This need for adaptation creates hurdles in industrial settings, where load changes are part of everyday operations.
Introducing the Hybrid Model
Leading this research initiative, Professor Taekyung Lee and Ph.D. candidate Jinyeong Yu aimed to address these limitations. Their study reveals a compelling integration of cutting-edge machine learning techniques and energy-based physical modeling. The model is designed to meet the challenges inherent in magnesium alloys by leveraging a combination of neural networks and physics-based assessments.
The neural network utilizes a comprehensive dataset comprising hysteresis loops—these graphs capture the stress-strain behaviors during numerous loading cycles of the AZ31 magnesium alloy. This dataset enables the network to learn the complexities of how the metal behaves under various stresses.
With this innovative model, manufacturers can anticipate fatigue life more accurately. The neural network reconstructs the loops for different conditions, thereby examining the energy dissipation during loading cycles closely linked to fatigue accumulation. The physics component further contextualizes these predictions, offering reliable estimates for the cycles until failure.
Flexible and Reliable Predictions
One of the most remarkable aspects of this hybrid model is its adaptability. Traditional methods often necessitate frequent manual readjustments to account for changing conditions, but the new model continuously learns and adapts using its neural network functionality. This self-adapting mechanism allows for increased flexibility across diverse loading directions without the burdensome need for manual intervention.
Implications for Industry
This breakthrough could revolutionize how manufacturers work with magnesium alloys, enhancing safety and durability in high-stakes environments. With improved predictive capability, companies can anticipate material fatigue more reliably, paving the way for the design of safer and lighter components.
As industries increasingly turn to advanced alloys, a model like this presents clear advantages, leading to more efficient manufacturing processes and better-performing vehicles and aircraft. The enhanced accuracy in fatigue life predictions not only promotes safety but could also result in significant cost savings for manufacturers over time.
Conclusion
The development of this hybrid predictive model signifies a significant leap forward in understanding and advancing the capabilities of magnesium alloys. By harnessing both machine learning and physical principles, researchers are unlocking new potential for creating safer, more effective materials in various applications.
Frequently Asked Questions
What is the primary function of the new hybrid model?
The model predicts the fatigue life of magnesium alloys under various stress conditions by integrating machine learning with physical principles.
How does this model improve upon traditional methods?
Unlike traditional methods, it does not require manual adjustments for different loading conditions and adapts automatically through machine learning.
Who are the key researchers behind the study?
The study was led by Professor Taekyung Lee and Ph.D. candidate Jinyeong Yu from Pusan National University.
What are magnesium alloys commonly used for?
Magnesium alloys are often utilized in vehicle and aircraft design due to their lightweight and strong characteristics.
What is the significance of accurately predicting fatigue life?
Accurate prediction helps ensure safety, reduces design costs, and enhances the durability of important components in various applications.
About The Author
Contact Dylan Bailey privately here. Or send an email with ATTN: Dylan Bailey as the subject to contact@investorshangout.com.
About Investors Hangout
Investors Hangout is a leading online stock forum for financial discussion and learning, offering a wide range of free tools and resources. It draws in traders of all levels, who exchange market knowledge, investigate trading tactics, and keep an eye on industry developments in real time. Featuring financial articles, stock message boards, quotes, charts, company profiles, and live news updates. Through cooperative learning and a wealth of informational resources, it helps users from novices creating their first portfolios to experts honing their techniques. Join Investors Hangout today: https://investorshangout.com/
The content of this article is based on factual, publicly available information and does not represent legal, financial, or investment advice. Investors Hangout does not offer financial advice, and the author is not a licensed financial advisor. Consult a qualified advisor before making any financial or investment decisions based on this article. This article should not be considered advice to purchase, sell, or hold any securities or other investments. If any of the material provided here is inaccurate, please contact us for corrections.