MANA Scientists Innovate Thermoelectric Material Development
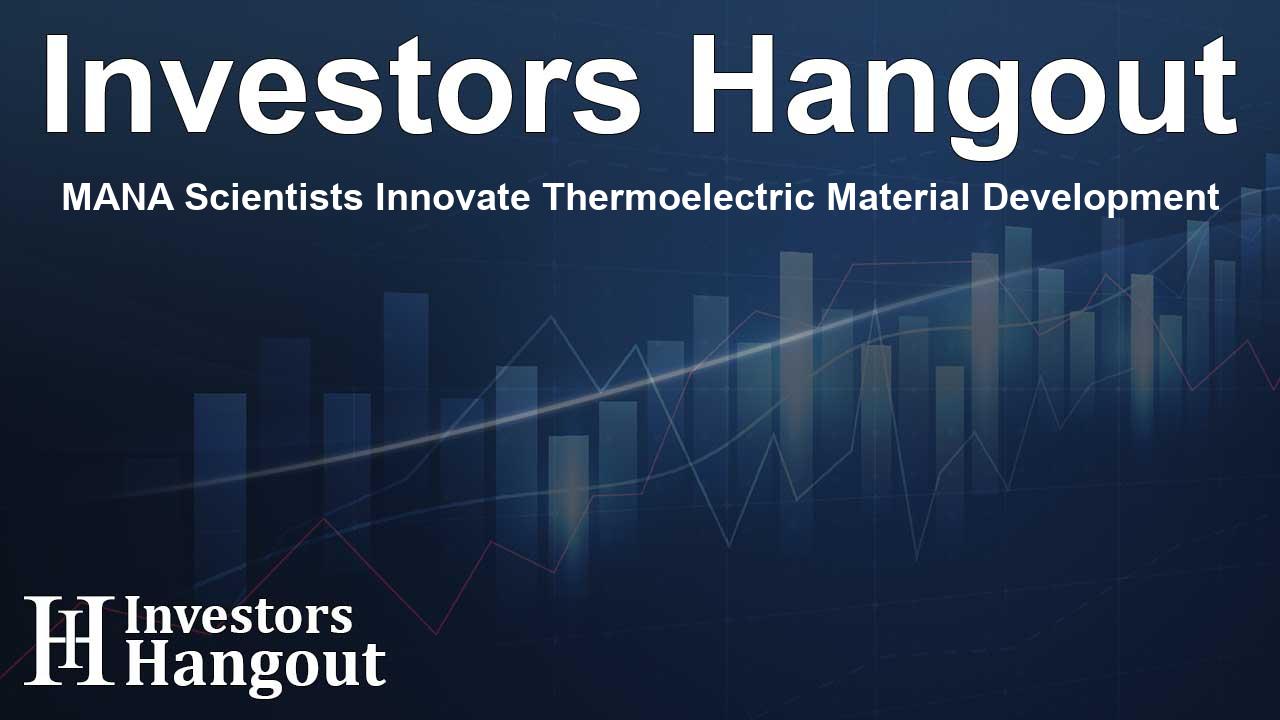
MANA Scientists Innovate Thermoelectric Material Development
The Research Center for Materials Nanoarchitectonics (MANA) has recently made significant strides in the realm of thermoelectric materials by employing advanced machine learning techniques. This innovative approach aims to expedite the discovery of kesterite-type thermoelectric materials, which play a crucial role in the advancement of efficient energy conversion technologies.
Understanding Kesterite-Type Thermoelectric Materials
Kesterite-type materials, such as Cu2ZnSnS4, are touted for their potential in thermoelectric applications, primarily for their ability to convert waste heat into usable electricity. One of the standout features of these materials is their non-toxic composition, made from plentiful and accessible elements. They exhibit a figure of merit (zT) of greater than 1 at various temperatures, specifically between 300 and 800K. At around 500K, these kesterite materials face a structural change that significantly influences their thermoelectric characteristics. Unfortunately, identifying the best manufacturing conditions is often a complex and lengthy process requiring multiple experimental iterations.
Accelerating the Research Process
To combat the time-consuming nature of traditional experimentation, MANA researchers leveraged the power of machine learning. Their commitment to innovation led them to optimize the sintering process in just four experimental cycles, leading to a remarkable enhancement in the thermoelectric performance of Cu2.125Zn0.875SnS4 by 60%. The research team was spearheaded by Dr. Cedric Bourges at the International Center for Young Scientists, alongside his colleagues from the Center for Basic Research on Materials.
Machine Learning in Materials Science
The groundbreaking study employed Active Learning with Bayesian Optimization (ALMLBO), a method that meticulously analyzes critical sintering parameters including heating rates, sintering temperatures, holding times, cooling rates, and applied pressure. By integrating these parameters with experimentally derived thermoelectric properties, the machine learning model was able to suggest optimized experimental conditions. This iterative approach continued until the team observed a stabilization in thermoelectric properties, marked by an improved zT value.
Data-Driven Success
The researchers initiated their project using data collected from 11 samples created through spark plasma sintering. This innovative technique involved combining powders of copper, zinc, tin, and sulfur under controlled atmospheric conditions. By pushing the boundaries of current knowledge, the ALMLBO model predicted sintering conditions that ultimately achieved a record maximum zT of 0.44 at 725K. This demonstrates how the fusion of machine learning with traditional materials science not only accelerates the research process but also enhances the optimization of complex material systems.
Potential Impact on Various Industries
The implications of this research extend far beyond just kesterite materials. The researchers anticipate that the successful approach can be applied to other material categories, which may spur rapid advancements in technologies such as photovoltaics, batteries, and electronics. Integrating machine learning into the materials discovery phase paves the way for innovation and efficiency, potentially leading to more sustainable energy solutions.
Frequently Asked Questions
What are kesterite-type thermoelectric materials?
Kesterite-type materials are non-toxic compounds capable of converting waste heat into electricity, notably Cu2ZnSnS4.
How does machine learning help in materials science?
Machine learning accelerates the discovery process by analyzing data and recommending optimal manufacturing conditions, significantly reducing experimentation time.
What was the improvement seen in the performance metrics?
The MANA team achieved a 60% enhancement in the thermoelectric performance of Cu2.125Zn0.875SnS4 through optimized sintering.
What is the significance of a zT value?
The zT value is a measure of thermoelectric efficiency, with higher values indicating better performance in waste heat conversion.
Which industries can benefit from this research?
This research impacts multiple industries, including photovoltaics, battery technology, and electronics, enabling sustainable energy innovations.
About Investors Hangout
Investors Hangout is a leading online stock forum for financial discussion and learning, offering a wide range of free tools and resources. It draws in traders of all levels, who exchange market knowledge, investigate trading tactics, and keep an eye on industry developments in real time. Featuring financial articles, stock message boards, quotes, charts, company profiles, and live news updates. Through cooperative learning and a wealth of informational resources, it helps users from novices creating their first portfolios to experts honing their techniques. Join Investors Hangout today: https://investorshangout.com/
Disclaimer: The content of this article is solely for general informational purposes only; it does not represent legal, financial, or investment advice. Investors Hangout does not offer financial advice; the author is not a licensed financial advisor. Consult a qualified advisor before making any financial or investment decisions based on this article. The author's interpretation of publicly available data presented here; as a result, they should not be taken as advice to purchase, sell, or hold any securities mentioned or any other investments. If any of the material offered here is inaccurate, please contact us for corrections.