Innovative Machine Learning Model Enhances Structural Safety
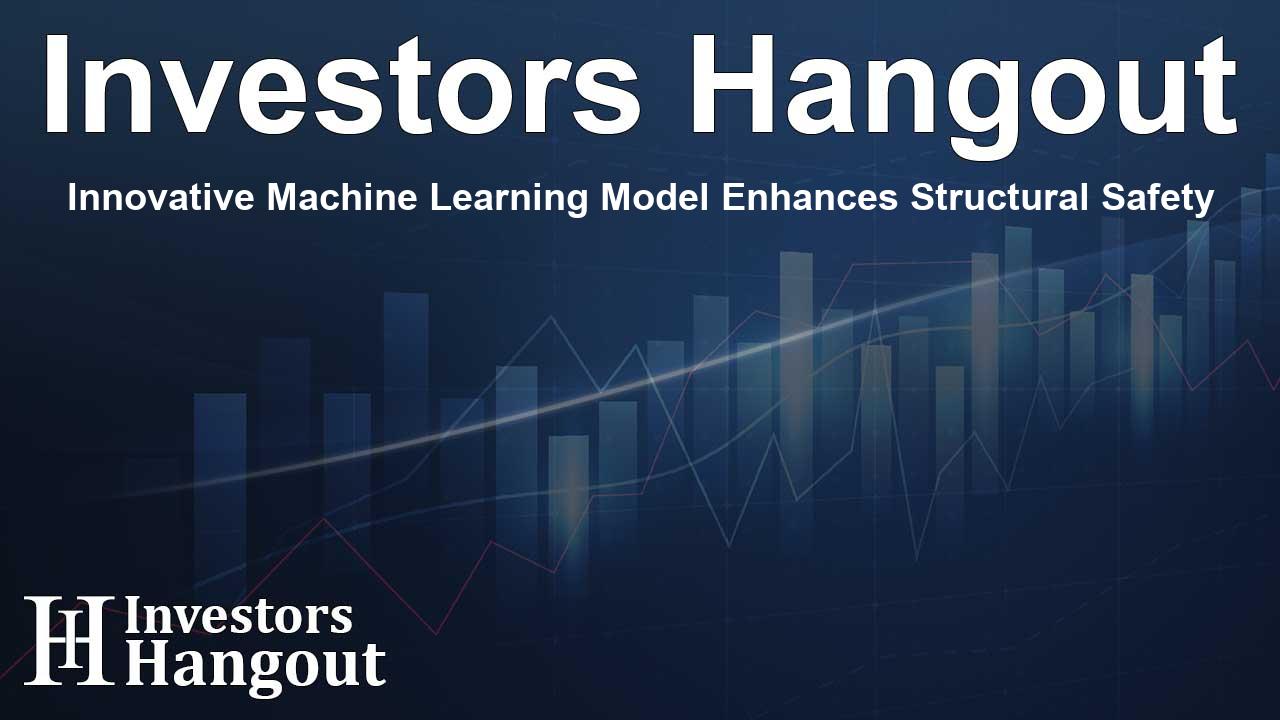
Transforming Structural Engineering with Machine Learning
No longer just a dream, enhanced structural integrity in constructions has become a reality with the introduction of machine learning techniques. Engineers seeking to improve safety and efficiency in buildings are now leveraging advanced computational methods. A recent breakthrough by a team at Seoul National University of Science and Technology has unveiled a promising model that predicts the strength of carbon fiber-reinforced steel columns, which are increasingly popular in modern construction.
The Power of Composite Materials
In the ever-evolving world of construction, the integration of concrete-filled steel tubes (CFST) with carbon fiber-reinforced polymers (CFRP) has shown immense potential. These composite structures combine the substantial load-bearing capacities of CFST with the lightweight and durability features of CFRP. As a result, this innovative material enhances not only the overall structural performance but also its longevity, minimizing maintenance needs over time.
Addressing Data Limitations
Despite the significant advantages presented by CFRP-strengthened CFST columns, engineers have faced challenges due to the limited data available for these materials. Insufficient data often leads to unpredictable performance when employing conventional predictive models. This is where the expertise of the research team at SeoulTech comes into play, aiming to overcome these hurdles using advanced machine learning techniques.
Development of the Hybrid Model
Under the leadership of Associate Professor Jin-Kook Kim, the research team has published a study detailing a novel hybrid machine learning model. This model is designed specifically to accurately predict the ultimate axial strength of CFRP-strengthened CFST columns, a crucial parameter for ensuring the structural integrity of buildings and infrastructure. The model was developed with a focus on generating reliable predictions critical for the construction industry.
Innovative Techniques for Data Generation
To tackle the challenge of insufficient real-world data for CFRP-strengthened structures, the team utilized generative adversarial networks (GANs) for synthesizing new data sets that mimic the characteristics of actual data. Dr. Kim highlighted the effectiveness of their chosen method, stating, "We employed a conditional tabular generative adversarial network, or 'CTGAN,' to generate new data with similar characteristics to real data." This approach ensured that their model had a robust training foundation.
Surpassing Conventional Models
Upon testing, the hybrid model combining Extra Trees (ET) technique with the Moth-Flame Optimization (MFO) algorithm exhibited remarkable performance against traditional empirical models. Dr. Kim pointed out that the MFO-ET model surpassed existing models in terms of accuracy, achieving lower error rates on essential metrics. This reliability was validated further through extensive reliability analyses, affirming the model's capability to deliver consistent predictions even in varied scenarios.
Real-World Applications and Future Implications
This cutting-edge model has the potential to revolutionize structural engineering practices. By enabling engineers to design safer and more efficient structures using CFRP-strengthened CFST columns, the model could become integral in constructing skyscrapers and retrofitting older buildings. Moreover, with climate change leading to harsher conditions, the corrosion-resistant features of CFRP materials make them formidable against the elements, promising a longer lifespan for critical infrastructure.
Accessing the Model and Its Benefits
To enhance the accessibility of this innovative predictive model, the research team has created a free web-based tool that allows users to make axial strength predictions easily. Engineers and construction professionals can utilize this tool from any device, simplifying the process of predicting structural integrity and bolstering construction safety.
A Significant Step Forward in Structural Assessment
The development of this hybrid model signifies a notable advancement in the assessment of CFRP-strengthened CFST columns. By combining machine learning with practical engineering applications, the research has opened new avenues for optimizing construction processes. Through reliable strength predictions, this research not only enhances safety and durability but also reduces operational costs for engineers and builders.
Frequently Asked Questions
What is the significance of CFRP-strengthened CFST columns?
CFRP-strengthened CFST columns enhance structural performance by combining lightweight and durable materials, improving both strength and longevity.
Who led the research at SeoulTech?
The research was led by Associate Professor Jin-Kook Kim, who focused on developing a predictive model for structural safety.
How does the new model generate data?
The team employed a conditional tabular generative adversarial network (CTGAN) to create synthetic data resembling real data for training their model.
Why is the hybrid model better than traditional models?
It offers superior accuracy with lower error rates and is capable of delivering consistent predictions across various conditions.
How can engineers access the new predictive model?
Engineers can access the web browser-based tool created by the research team for free, allowing them to make predictions without software installation.
About Investors Hangout
Investors Hangout is a leading online stock forum for financial discussion and learning, offering a wide range of free tools and resources. It draws in traders of all levels, who exchange market knowledge, investigate trading tactics, and keep an eye on industry developments in real time. Featuring financial articles, stock message boards, quotes, charts, company profiles, and live news updates. Through cooperative learning and a wealth of informational resources, it helps users from novices creating their first portfolios to experts honing their techniques. Join Investors Hangout today: https://investorshangout.com/
Disclaimer: The content of this article is solely for general informational purposes only; it does not represent legal, financial, or investment advice. Investors Hangout does not offer financial advice; the author is not a licensed financial advisor. Consult a qualified advisor before making any financial or investment decisions based on this article. The author's interpretation of publicly available data presented here; as a result, they should not be taken as advice to purchase, sell, or hold any securities mentioned or any other investments. If any of the material offered here is inaccurate, please contact us for corrections.