Innovative AI Hardware Solutions via Silicon Photonics Revolution
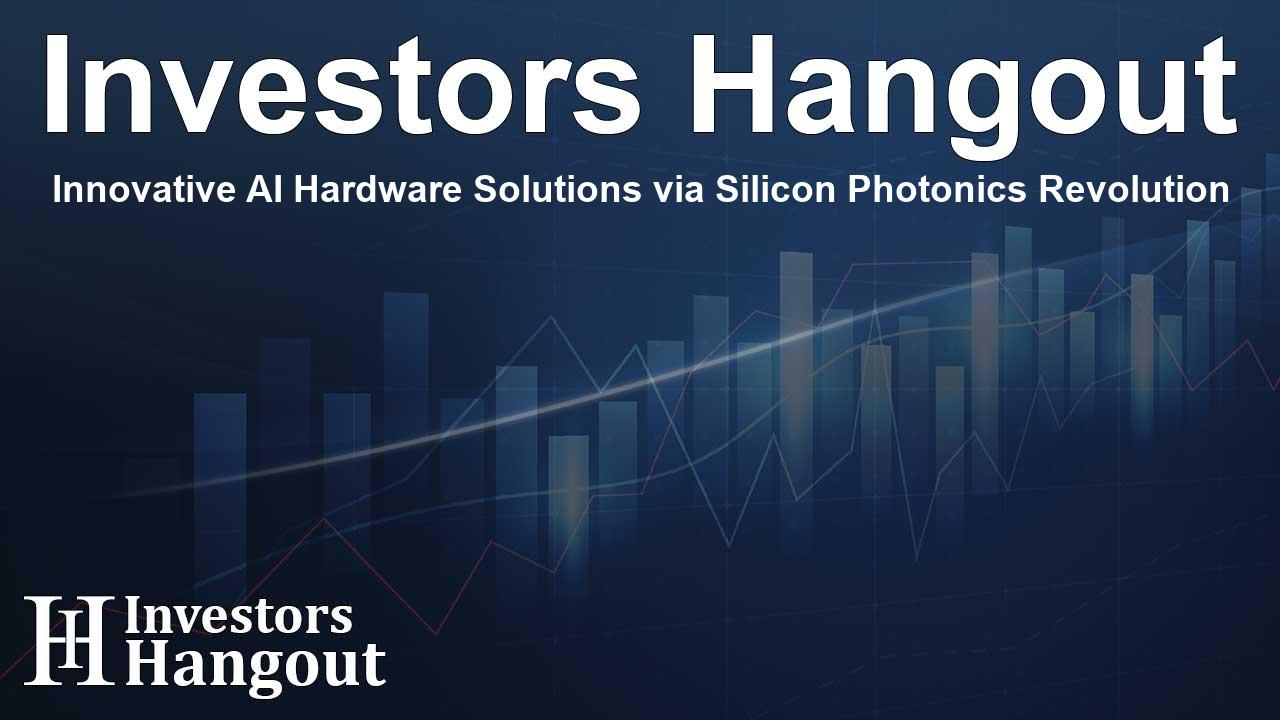
Transforming the Future of AI with Silicon Photonics
Researchers have unveiled an innovative hardware platform designed for AI accelerators that efficiently manages heavy workloads while optimizing energy consumption.
The Rise of AI and Its Challenges
Artificial Intelligence (AI) has greatly influenced various sectors, driven by advancements in deep learning technology and the analysis of large datasets. This evolution of AI demands immense computational power for the development and training of models. Traditionally, the AI infrastructure relies heavily on graphical processing units (GPUs). However, the vast computational requirements and high energy costs associated with GPU operations pose significant hurdles for widespread AI adoption.
Adoption of Photonic Integrated Circuits
A groundbreaking study has been published in the IEEE Journal of Selected Topics in Quantum Electronics, highlighting an advanced AI acceleration platform that leverages photonic integrated circuits (PICs). These circuits promise to offer remarkable scalability and energy efficiency when compared to traditional GPU-based architectures. Led by Dr. Bassem Tossoun from Hewlett Packard Labs, the research illuminates how PICs, built with III-V compound semiconductors, are capable of executing AI workloads with great efficiency.
Unlike standard AI hardware that operates on electronic distributed neural networks (DNNs), photonic AI accelerators harness optical neural networks (ONNs). These networks operate at the speed of light, significantly minimizing energy loss during data processing.
Insights from the Research
Dr. Tossoun expresses the potential of silicon photonics: "While silicon photonics are easy to manufacture, their scalability for intricate integrated circuits remains a challenge. Our device platform serves as a foundation for photonic accelerators, offering energy efficiency and scalability that surpasses current technologies." The team's approach included a unique heterogeneous integration technique to create the hardware.
This involved using silicon photonics combined with III-V compound semiconductors, effectively integrating lasers and optical amplifiers, thereby reducing optical losses while enhancing scalability.
The Fabrication Process Explained
The creation of this innovative hardware began with silicon-on-insulator (SOI) wafers that feature a 400 nm-thick silicon layer. During the fabrication process, various techniques were applied, including lithography, dry etching, and doping for metal oxide semiconductor capacitor (MOSCAP) components and avalanche photodiodes (APDs). Following this, selective growth of silicon and germanium was executed to develop absorption, charge, and multiplication layers of the APD. Furthermore, III-V compound semiconductors, such as InP or GaAs, were integrated onto the silicon platform through die-to-wafer bonding. A thin layer of gate oxide (Al?O? or HfO?) was included to amplify device effectiveness, with a thick dielectric layer applied for encapsulation and thermal stability.
This pioneering photonic platform can achieve comprehensive wafer-scale integration of necessary components to construct an optical neural network on a single chip. These components include on-chip lasers, high-speed photodetectors, energy-efficient modulators, and non-volatile phase shifters. Looking forward, this photonic platform is set to enhance datacenter capabilities to manage increasing AI workloads, addressing numerous optimization challenges that exist within current systems.
A Sustainable Approach to AI Acceleration
The introduction of this hardware platform is a significant resolve to the computational and energy dilemmas faced in the AI sector, paving the way for robust and sustainable AI accelerator solutions in the upcoming times!
Frequently Asked Questions
What is silicon photonics?
Silicon photonics is a technology that uses silicon as the medium for transmitting data as light signals, which offers potential advantages in speed and energy efficiency.
How do optical neural networks work?
Optical neural networks process information using light, allowing for faster data transmission and lower energy consumption compared to traditional electronic networks.
What are the main benefits of this new AI accelerator platform?
This platform enhances scalability and energy efficiency, making it ideal for handling large AI workloads effectively.
Who led the research on this new hardware platform?
The research was spearheaded by Dr. Bassem Tossoun, a Senior Research Scientist at Hewlett Packard Labs.
What challenges does this technology address in AI?
This technology primarily addresses the growing demand for computational power and the associated energy challenges by offering a more efficient hardware solution.
About The Author
Contact Kelly Martin privately here. Or send an email with ATTN: Kelly Martin as the subject to contact@investorshangout.com.
About Investors Hangout
Investors Hangout is a leading online stock forum for financial discussion and learning, offering a wide range of free tools and resources. It draws in traders of all levels, who exchange market knowledge, investigate trading tactics, and keep an eye on industry developments in real time. Featuring financial articles, stock message boards, quotes, charts, company profiles, and live news updates. Through cooperative learning and a wealth of informational resources, it helps users from novices creating their first portfolios to experts honing their techniques. Join Investors Hangout today: https://investorshangout.com/
The content of this article is based on factual, publicly available information and does not represent legal, financial, or investment advice. Investors Hangout does not offer financial advice, and the author is not a licensed financial advisor. Consult a qualified advisor before making any financial or investment decisions based on this article. This article should not be considered advice to purchase, sell, or hold any securities or other investments. If any of the material provided here is inaccurate, please contact us for corrections.