Exploring the Impact of RAG on Generative AI Utilization
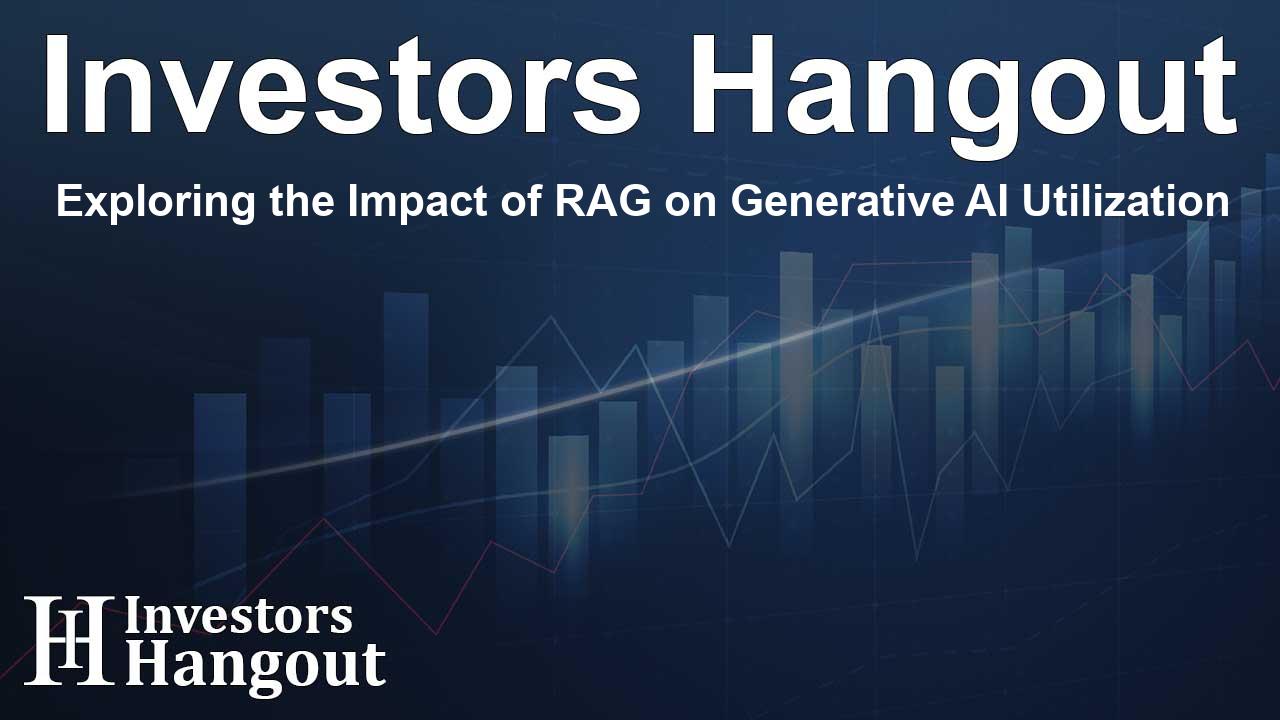
Understanding the Role of RAG in Generative AI
The landscape of generative AI (GenAI) is rapidly evolving, and organizations are increasingly integrating advanced methodologies to enhance information management. A recent comprehensive survey delves into how retrieval-augmented generation (RAG) environments can significantly improve the handling of information within enterprises. With the growing reliance on large language models (LLMs), businesses are now more than ever keen on employing tools that not only streamline processes but also foster data integrity and effective governance.
Challenges Faced by Organizations in AI Integration
As companies embrace the potential of generative AI, various challenges emerge. Chief among them are concerns over data security, consistency, and the quality of insights generated. It's vital to establish robust data governance frameworks to address these issues effectively. Businesses are realizing that the transition to AI requires an understanding of data handling intricacies, ensuring employees are well-equipped to utilize these technologies safely and effectively.
Data Governance as a Priority
The new survey highlights that 71% of respondents feel apprehensive regarding the output produced by GenAI technologies, particularly focusing on the risks posed by incorrect data and bias in outcomes. Organizations are starting to recognize that in the rush to adopt AI solutions, they must prioritize data governance to mitigate these risks.
The Pervasiveness of LLMs in Organizations
Interestingly, despite the challenges, the use of LLMs is on the rise. About 85% of executives surveyed reported either exploring or testing LLMs for their applications. From generating content to discovering knowledge, LLM implementations are expected to become a staple component of organizational processes. Companies anticipate significant productivity gains and quicker access to insights, helping knowledge workers perform their tasks more efficiently.
The Promise of RAG Systems
To address these nuanced needs, many organizations are turning to RAG systems. About 29% of companies are currently implementing RAG solutions to effectively bridge the gap between existing data infrastructures and generative AI capabilities. This strategic move is recognized as essential in safeguarding the integrity of information while also enhancing contextual accuracy.
Bridging the Gap with Knowledge Graphs
One key element cited for the success of RAG systems is the incorporation of knowledge graphs designed to leverage structured and unstructured data. This architectural shift enables organizations to remove barriers that commonly stifle generative AI success, ensuring that the insights drawn are both actionable and reliable.
Real-Time Actionability and Benefits
Organizations are increasingly aware that the strategic integration of RAG can lead to more actionable insights closer to real-time usage. This requires a delicate balance of technology and governance to ensure that generated insights are not only relevant but also transformative. Such advancements can significantly enhance decision-making processes, improve customer experiences, and lead to overall operational efficiency.
The Future Landscape of Generative AI
As companies continue to invest in generative AI technologies, the assurance lies in building a solid foundation. Experts like Andreas Blumauer from Graphwise stress the importance of establishing rigorous data quality controls. Businesses must guard against the dangers posed by inaccurate data and misinformed conclusions that could lead to costly mistakes or reputational harm.
Ultimately, the journey into generative AI requires thoughtful integration of technologies like RAG and knowledge graphs, ensuring alignment between AI capabilities and strategic business goals. Organizations that successfully navigate this landscape can elevate their knowledge management, making it a competitive advantage.
Frequently Asked Questions
What is generative AI?
Generative AI refers to algorithms that can generate text, images, or other media in response to prompts, utilizing technologies like large language models.
How does RAG enhance AI performance?
RAG improves the performance of AI systems by integrating external data sources, ensuring that the insights generated are accurate and contextually relevant.
What are knowledge graphs?
Knowledge graphs are structured representations of information that facilitate better data connections and improve the usability of AI systems.
Why is data governance important in AI?
Data governance ensures that the data used in AI applications is accurate, secure, and compliant with regulations, reducing risks associated with AI outcomes.
How can organizations leverage AI for better productivity?
By adopting generative AI tools and RAG systems, organizations can streamline workflows, enhance decision-making processes, and foster improved customer experiences.
About The Author
Contact Owen Jenkins privately here. Or send an email with ATTN: Owen Jenkins as the subject to contact@investorshangout.com.
About Investors Hangout
Investors Hangout is a leading online stock forum for financial discussion and learning, offering a wide range of free tools and resources. It draws in traders of all levels, who exchange market knowledge, investigate trading tactics, and keep an eye on industry developments in real time. Featuring financial articles, stock message boards, quotes, charts, company profiles, and live news updates. Through cooperative learning and a wealth of informational resources, it helps users from novices creating their first portfolios to experts honing their techniques. Join Investors Hangout today: https://investorshangout.com/
The content of this article is based on factual, publicly available information and does not represent legal, financial, or investment advice. Investors Hangout does not offer financial advice, and the author is not a licensed financial advisor. Consult a qualified advisor before making any financial or investment decisions based on this article. This article should not be considered advice to purchase, sell, or hold any securities or other investments. If any of the material provided here is inaccurate, please contact us for corrections.