AI-Powered Anomaly Detection and Spotting Sophisticated Document Alterations
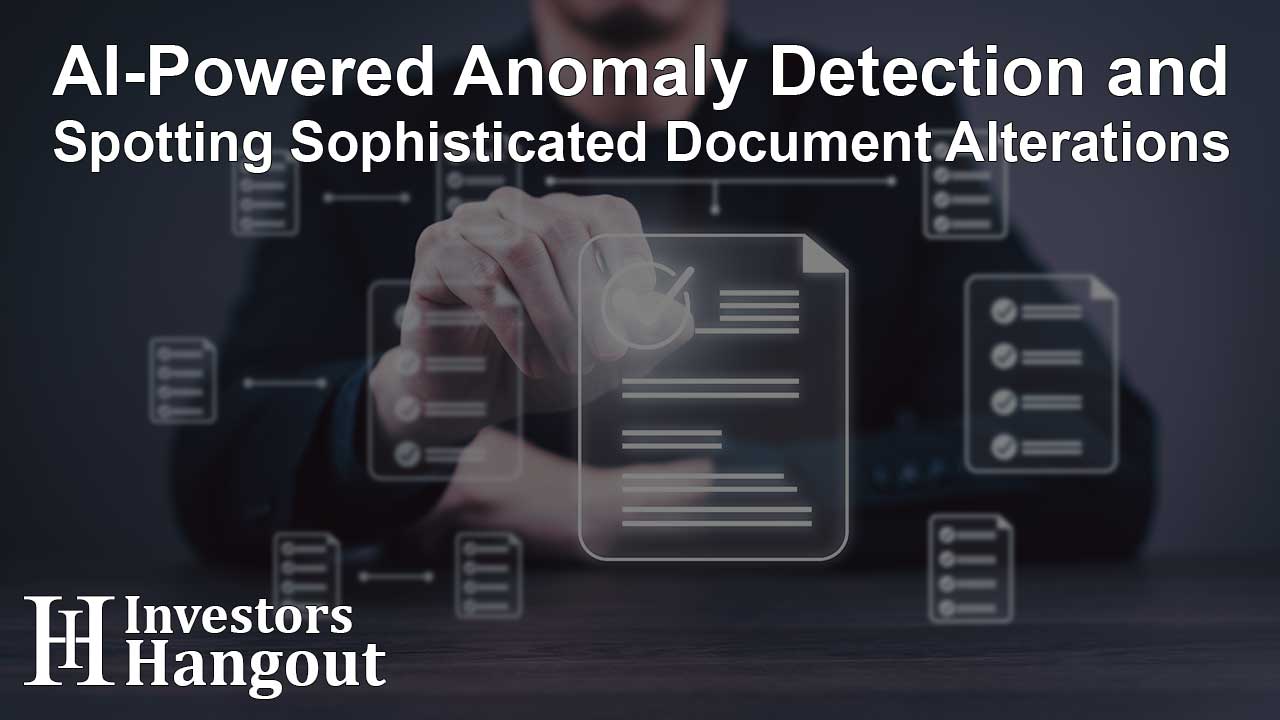
The Importance of Authentic Documents in the Digital Age
Do you know what is common between financial transactions, legal proceedings, English learning, and international travel? The answer is authentic documents.
For centuries, the verification of these crucial papers relied on human expertise and evolving security features. However, the digital revolution has altered this landscape, introducing new vulnerabilities while simultaneously offering groundbreaking solutions.
In the digital age, document fraud has evolved into a sophisticated menace, threatening the integrity of financial systems, government processes, educational institutions, and personal identities. As fraudsters employ increasingly advanced techniques, traditional detection methods struggle to keep pace.
This escalating challenge has necessitated a paradigm shift in fraud prevention strategies. Artificial Intelligence (AI) emerges as a formidable ally in this high-stakes battle, offering unprecedented capabilities in anomaly detection.
This blog post will talk about how AI is revolutionizing authenticity checks in documents. Moreover, it will also give you reasons to embrace this disruption at the drop of a hat.
Understanding AI-Powered Anomaly Detection
Fraud document detection, explained in simple terms, refers to analyzing documents for signs of forgery or manipulation. Anomaly detection, notes AU10TUX, identifies deviations from expected document patterns or characteristics. AI enhances this process through rapid, multi-dimensional analysis.
Machine learning algorithms, particularly unsupervised learning models, excel at establishing baseline document characteristics and flagging deviations.
The role of machine learning in improving detection accuracy is crucial. Through iterative training on vast datasets, these systems refine their ability to distinguish genuine anomalies from benign variations. Ensemble methods, combining multiple AI models, reduce false positive rates while maintaining high sensitivity to fraud indicators.
Convolutional Neural Networks (CNNs), for example, detect pixel-level alterations in images. IBM states that these methods are inspired by the workings of the human brain and are like image analysis experts. They process pictures piece by piece, mimicking how we see, to identify and categorize objects within them.
AI's adaptive learning capabilities enable real-time updates to detection parameters. They ensure that the system evolves in tandem with emerging fraud techniques, offering businesses a robust, future-proof solution for document verification challenges.
Key Features of AI Document Checks
An automated documents verification tool employs a suite of sophisticated features to combat fraud. Pixel-level analysis leverages deep learning algorithms to detect microscopic alterations. It compares document images against authenticated templates with sub-pixel accuracy.
Metadata examination can also scrutinize hidden document properties. According to Case IQ, digital documents contain metadata that can provide information on the authorship, timing, and specific modifications made to the document.
Moving on, cross-referencing capabilities tap into extensive, continuously updated databases. This enables real-time validation of document authenticity across global jurisdictions.
Adaptive learning, a cornerstone of fraud document detection, allows systems to evolve dynamically. Generative adversarial networks (GANs) simulate potential fraud techniques, and train detection models to recognize emerging threats proactively.
These features synergize to create a robust, multi-layered defense against document fraud. However, for the sake of operational feasibility, many businesses outsource risk assessment and fraud detection tasks to third-party experts.
Many cutting-edge platforms offer biometric modalities and data analytics as fraud detection methods. The majority of these systems proudly feature in-house, AI-powered systems trained to identify trends and irregularities linked to fraudulent documents or activity.
Some Real-World Use Cases of Fraud Detection
In the financial arena, neural networks analyze the micro-features of checks and contracts. They detect subtle inconsistencies in paper quality, ink distribution, and signature dynamics. These systems boast accuracy rates exceeding 99%, significantly outperforming traditional methods.
Government agencies employ multi-modal biometric verification, integrating facial recognition with document analysis. Advanced algorithms cross-reference ID elements against secure databases in milliseconds. According to ResearchGate, anomalies can then be flagged in hologram patterns or RFID chip data.
Healthcare institutions leverage natural language processing to scrutinize prescription syntax and frequency. According to the National Institutes of Health, convolutional neural networks examine medical records for unauthorized image alterations. These AI systems can identify anomalies in medication dosages or treatment histories with remarkable precision.
In the legal sector, machine learning models analyze document metadata, verifying timestamps and digital signatures. These systems can detect subtle linguistic inconsistencies indicative of fraudulent modifications, ensuring the integrity of critical legal documents.
Across industries, AI-driven anomaly detection not only enhances security but also streamlines operations, reducing verification times while maintaining unparalleled accuracy.
FAQs
Q1: How does AI-powered anomaly detection differ from traditional document verification methods?
A: AI-powered anomaly detection uses machine learning algorithms to analyze documents at a micro-level, detecting subtle inconsistencies that human eyes might miss. It can process vast amounts of data quickly, adapt to new fraud techniques in real time, and reduce false positives while maintaining high accuracy.
Q2: Can AI-powered document verification systems be fooled by sophisticated forgeries?
A: While no system is infallible, AI-powered systems are continuously learning and adapting. They use advanced techniques, like generative adversarial networks, to anticipate and recognize new fraud methods. This adaptability makes them significantly more robust against sophisticated forgeries than traditional methods.
Q3: Is AI-powered document verification suitable for small businesses?
A: Yes, AI-powered document verification can benefit businesses of all sizes. Many providers offer scalable solutions that can be tailored to specific needs and budgets. Efficiency and accuracy improvements can lead to significant cost savings and risk reductions for small businesses.
To sum up, as we progress into an era of sophisticated digital interactions, the importance of reliable document verification extends beyond mere fraud prevention. It becomes a pillar of trust in our digital society, facilitating smoother transactions, enhancing public safety, and fostering innovation.
The advent of AI-powered anomaly detection represents not just a technological advancement, but a shift towards a more secure and efficient global ecosystem.
As these systems continue to evolve, they promise to redefine the boundaries of what's possible in document security. They wield power to revolutionize how we approach identity verification, contractual agreements, and information authenticity in the digital age.
About The Author
Contact Ryan Hughes privately here. Or send an email with ATTN: Ryan Hughes as the subject to contact@investorshangout.com.
About Investors Hangout
Investors Hangout is a leading online stock forum for financial discussion and learning, offering a wide range of free tools and resources. It draws in traders of all levels, who exchange market knowledge, investigate trading tactics, and keep an eye on industry developments in real time. Featuring financial articles, stock message boards, quotes, charts, company profiles, and live news updates. Through cooperative learning and a wealth of informational resources, it helps users from novices creating their first portfolios to experts honing their techniques. Join Investors Hangout today: https://investorshangout.com/