Machine Learning Model Enables Researchers to Pinp
Post# of 138
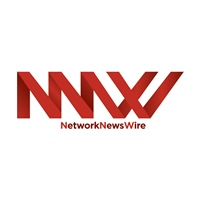
Researchers have designed a machine learning model that can identify individuals suffering from PTSD by conducting an analysis of text data with 80% accuracy. Post-traumatic stress disorder (PTSD) is a mental health condition that is triggered by a traumatic event which an individual may witness or experience.
This machine learning model may, in the future, be used as an inexpensive and accessible screening method to help physicians detect and diagnose post-traumatic stress disorder or other mental conditions, via telehealth platforms. Jeff Sawalha, a PhD candidate specializing in psychiatry at the University of Alberta, led this project. He carried out an analysis of text obtained from a dataset developed by a researcher at the Institute for Creative Technologies in USC. This sentiment analysis involved categorizing a huge body of data comprised of interview responses gathered over video conferencing calls with individuals who did and did not suffer from PTSD.
Sawalha stated that the objective of the study was to see whether the model could accurately identify people with post-traumatic stress disorder, based on the emotional content of the data. The researchers succeeded in determining the people with PTSD using scores that indicated whether these individuals’ speeches primarily featured negative or neutral responses.
Sawalha explained that this was in line with literature on post-traumatic stress disorder and emotion, noting that while some individuals had no issue expressing their negative emotions, others tended to numb their emotions, be neutral and refrain from saying too much.
The coauthor of the study, Russ Greiner of the Department of Computing Science, highlighted that this process to identify individuals with PTSD was complicated, given that the abundance in simple phrases could be hard to categorize. Greiner is scientific director for the Alberta Machine Intelligence Institute.
However, the model’s success in identifying those with post-traumatic stress disorder solely from text data posits that similar models can be applied to other sets of data to identify individuals suffering from other mental health conditions. Sawalha noted that machine learning also made it easier to learn the complex patterns that could help differentiate individuals who suffered from a specific mental illness.
The researchers are now focused on collaborating with partners at the University of Alberta in order to find out whether integrating other data types, such as motion or speech, could improve the model. They also hope to analyze mental health conditions such as schizophrenia and neurological disorders such as Alzheimer’s, which have a language component.
As the diagnostic tools for various mental health conditions improve, there is also a need to speed up the development of new lines of treatment for these illnesses. Entities such as Silo Pharma Inc. (OTCQB: SILO) are responding to this need, and breakthroughs are imminent in the coming years.
NOTE TO INVESTORS: The latest news and updates relating to Silo Pharma Inc. (OTCQB: SILO) are available in the company’s newsroom at https://ibn.fm/SILO
Please see full terms of use and disclaimers on the PsychedelicNewsWire website applicable to all content provided by PNW, wherever published or re-published: https://www.PsychedelicNewsWire.com/Disclaimer

