What must happen Before datalock & evaluation?
Post# of 72437
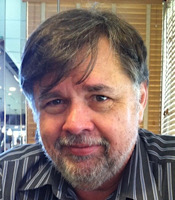
Clinical Data Collection, Cleaning and Verification in Anticipation of Database Lock
Practices and Recommendations
Vera Pomerantseva & Olga Ilicheva
Pharmaceutical Medicine volume 25, pages 223–233 (2011)
Abstract
The gathering of data is central to the evaluation of new and approved drugs and every stage of trial design and data collection involves a set of cleaning and validation procedures to ensure the validity of the data. Preparation for data cleaning and validation must begin before the actual data are entered into the database and continue until the end of the data collection process. Data collection instruments have a big impact on the design of data management activities in terms of possible error types and their prevention methods, data load timelines and data verification options. Quality control must be applied at every stage of data handling and the data error rate should be estimated and recorded in the data management master file. The acceptable error rate for a database varies in the industry, but a popular choice is 0.5% overall, with 02–0.1% for critical variables and 0.22–1% for non-critical variables. Data management plans or other referenced documents should define data entry and processing plans. The minimum standard requirement is to document any findings and corrective actions, perform at least one quality assessment before database lock, use statistically appropriate sample study population sizes for decision making and determine separate acceptable error rates for primary and secondary safety and efficacy variables. Best practice recommendations for more complex procedures include: (i) performing quality control on whole-study populations (not just sample sizes), especially for variables that are related to primary and secondary endpoint evaluations; (ii) comparing trial data at multiple timepoints; (iii) monitoring data by site to detect which data differ significantly, so that appropriate corrective actions can be taken; and (iv) developing quantitative methods to measure data quality. The data manager has to ensure completion of all visits and assessments by all study subjects before initiating database lock. Once all of the data have been transferred and captured in the database, final cleaning, reconciliation and verification activities can be performed. All queries must be clarified. Having multiple incremental soft locks at certain timepoints of the study is a very effective tool for ensuring valid data. The lock of a database is a very important milestone in a study and once the final database lock has occurred, no further changes can be made without special permission. Before everyone moves on to new projects and forgets details, managers should allocate time to make sure that the study documentation is complete and that feedback from the study is recorded. Right after the lock is a good time to review the case report form fields that caused difficulties, or to add notes to the file to record any unusual circumstances that may have occurred. This is also a perfect time to review the metrics from the study such as the total number of pages, total number of discrepancies, percentages of discrepancies resolved inhouse, average time to resolve queries and time from the last query until study database lock. The locked database should remain in the system for at least 3 months before it is archived.
This is a preview of subscription content, access via your institution.
References
1.
Wayne Eckerson. Who ensures clean, consistent data. The Data Warehouse Institute, 2009 Sep [online]. Available from URL: http://www.sas.com/resources/asset/104222_1009.pdf [Accessed 2011 Apr 8]
Google Scholar
2.
Society for Clinical Data Management. Good clinical data management practices (GCDMP). 4th version. Milwaukee (WI): Society for Clinical Data Management, 2007
Google Scholar
3.
Institute of Medicine. Assuring data quality and validity in clinical trials for regulatory decision making: workshop report [online]. Available from URL: http://www.iom.edu/Reports/1999/Assuring-Data...eport.aspx [Accessed 2011 June 27]

