AI Could Help Find EV Charging Infrastructure Gaps
Post# of 869
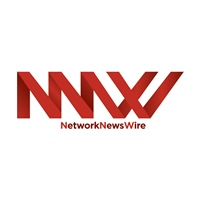
Plenty of drivers would like to switch to electric vehicles (“EVs”) because they produce zero emissions at the tailpipe and are generally cheaper to maintain and fuel over the lifetime of the vehicle. However, the current network of public charging stations doesn’t inspire much confidence in prospective EV drivers, with most drivers being wary of running out of charge without a charging station in sight. The fact that most of the charging stations are largely run by independent entities with no connection to each other and that don’t share data makes it difficult to build a reliable network of charging stations.
However, researchers have employed both technology and community engagement to help address this problem. Using artificial intelligence (“AI”) trained to analyze EV charging station reviews left by thousands of drivers on charge station locator apps, researchers can accurately identify regions with inadequate or even out-of-service charging stations. The researchers reported their results in the “Patterns” journal. Omar Asensio, the principal investigator and an assistant professor in the School of Public Policy at the Georgia Institute of Technology, says that although companies are spending huge amounts of dollars to develop EV charging infrastructure, the industry lacks a concrete understanding of how adequately this investment is serving members of the public.
In the past, previous studies designed to evaluate how well public EV charging stations were serving the public relied on infrequent self-reported surveys and proved to be costly. Machine learning, on the other hand, has allowed researchers to reduce associated costs while simultaneously providing real-time standardized data. To achieve this feat, Asensio and his team trained their algorithm with customer reviews from 12,720 charging stations in the United States, tailoring it to the lingo used in the electric vehicle space.
Their AI classified the reviews into eight categories: functionality, availability, cost, location, dealership, user interaction, service time and range anxiety. According to Asensio, the algorithm ultimately attained 91% accuracy and high learning efficiency, exceeding the performance of human experts in time. The artificial intelligence allowed him and his team to discover that micropolitan locations (places having 10,000 to 50,000 inhabitants) could be generally undeserved and had more frequent station availability reports. These communities are mostly in states within the West and Midwest such as Utah, Nebraska, Oregon and South Dakota.
With AI trained to read reviews in play, policy analysis will be much quicker, making infrastructure management much easier. Lead investigator Asensio and his team hope their work with machine learning can allow more extensive studies on not just meeting consumer needs but also social equity, especially in regards to access to energy services.
The EV space is set for even more innovation, as Net Element (NASDAQ: NETE), the owner of the Aptito restaurant management platform, is poised to complete a merger that will make it part of an EV manufacturer based in California.
NOTE TO INVESTORS: The latest news and updates relating to Net Element (NASDAQ: NETE) are available in the company’s newsroom at http://ibn.fm/NETE
Please see full terms of use and disclaimers on the Green Car Stocks website applicable to all content provided by GCS, wherever published or re-published: https://www.GreenCarStocks.com/Disclaimer

