How Artificial Intelligence Is Accelerating EV Bat
Post# of 1081
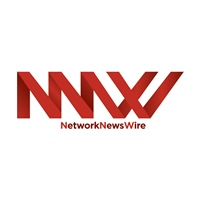
Although electric vehicles (“EVs”) are poised to replace internal combustion engine vehicles over the next couple of decades, EV technology is constantly evolving. Unlike conventional vehicles, EVs are powered by rechargeable lithium-ion batteries, and EV makers, as well as battery manufacturers, have been working to improve and refine battery technology. The ultimate goal is to develop an EV battery pack that can provide adequate range and charge rapidly without degrading.
Researchers are now turning to artificial intelligence (“AI”) to help accelerate battery development. However, for these battery building AIs to be effective, they need sufficient data, something that is quite scarce in the battery-technology space. To generate the much-needed data, a team of researchers from MIT, Stanford and the Toyota Research Institute used half a dozen machines to charge and discharge batteries numerous times daily.
The six refrigerator-sized machines, which held around 100 lithium-ion batteries each, charged and discharged the batteries dozens of times per day, generating tons of data on battery performance. The data was then fed to an AI to teach it how to build a more efficient battery. Ordinarily, it would take months of charging and discharging the batteries to collect such data, and it could only be fed to the AI once the battery started degrading.
However, the researchers were able to cut that expected time down drastically — to hours — allowing them to predict a lithium-ion battery’s lifetime performance after just a few hours of collecting data, all while the battery is in peak condition. In a paper published in “Nature,” the researchers described an AI that was able to discover the most efficient method for fast charging a lithium-ion battery in 10 minutes after only a month. According to Stanford material scientist William Chueh, that discovery would have taken an estimated two years without AI.
Artificial intelligence is also being used to find molecules that could improve the performance of a battery’s electrolyte. Ian Foster, the director of the data science and learning division at Argonne National Library, and his colleagues have been building a database of such molecules. The team from Argonne published a paper in late 2019 detailing how they used a database of 133,000 organic molecules to train an AI on how to find the most suitable molecules.
Training the AI with a smaller data set will enable it to explore a much larger database, one containing 166 billion potential materials, for example, with ease and efficiency. However, Foster cautions, it will still take years before what researchers discover via AI is installed in millions of EVs.
As the industry undergoes rapid evolution, so are companies operating within it. For instance, Net Element (NASDAQ: NETE), a payment-as-a-service firm, is merging with an EV manufacturer (Mullen Technologies Inc.), creating a new entity designed to be a strong player in the space.
NOTE TO INVESTORS: The latest news and updates relating to Net Element (NASDAQ: NETE) are available in the company’s newsroom at http://ibn.fm/NETE
Please see full terms of use and disclaimers on the Green Car Stocks website applicable to all content provided by GCS, wherever published or re-published: https://www.GreenCarStocks.com/Disclaimer

