here is a other article on why the driver of a sel
Post# of 40989
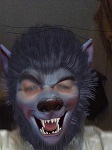
A new study finds a potential risk with self-driving cars: failure to detect dark-skinned pedestrians
The findings speak to a bigger problem in the development of automated systems: algorithmic bias.
By Sigal Samuel Updated Mar 6, 2019, 10:50am EST
The list of concerns about self-driving cars just got longer.
In addition to worrying about how safe they are, how they’d handle tricky moral trade-offs on the road, and how they might make traffic worse, we also need to worry about how they could harm people of color.
If you’re a person with dark skin, you may be more likely than your white friends to get hit by a self-driving car, according to a new study out of the Georgia Institute of Technology. That’s because automated vehicles may be better at detecting pedestrians with lighter skin tones.
The authors of the study started out with a simple question: How accurately do state-of-the-art object-detection models, like those used by self-driving cars, detect people from different demographic groups? To find out, they looked at a large dataset of images that contain pedestrians. They divided up the people using the Fitzpatrick scale, a system for classifying human skin tones from light to dark.
The researchers then analyzed how often the models correctly detected the presence of people in the light-skinned group versus how often they got it right with people in the dark-skinned group.
The result? Detection was five percentage points less accurate, on average, for the dark-skinned group. That disparity persisted even when researchers controlled for variables like the time of day in images or the occasionally obstructed view of pedestrians.
“The main takeaway from our work is that vision systems that share common structures to the ones we tested should be looked at more closely,” Jamie Morgenstern, one of the authors of the study, told me.
The report, “Predictive Inequity in Object Detection,” should be taken with a grain of salt. It hasn’t yet been peer-reviewed. It didn’t test any object-detection models actually being used by self-driving cars, nor did it leverage any training datasets actually being used by autonomous vehicle manufacturers. Instead, it tested several models used by academic researchers, trained on publicly available datasets. The researchers had to do it this way because companies don’t make their data available for scrutiny — a serious issue given that this a matter of public interest.
That doesn’t mean the study isn’t valuable. As Kate Crawford, a co-director of the AI Now Research Institute who was not involved in the study, put it on Twitter: “In an ideal world, academics would be testing the actual models and training sets used by autonomous car manufacturers. But given those are never made available (a problem in itself), papers like these offer strong insights into very real risks.”
Algorithms can reflect the biases of their creators
The study’s insights add to a growing body of evidence about how human bias seeps into our automated decision-making systems. It’s called algorithmic bias.
The most famous example came to light in 2015, when Google’s image-recognition system labeled African Americans as “gorillas.” Three years later, Amazon’s Rekognition system drew criticism for matching 28 members of Congress to criminal mugshots. Another study found that three facial-recognition systems — IBM, Microsoft, and China’s Megvii — were more likely to misidentify the gender of dark-skinned people (especially women) than of light-skinned people.
Since algorithmic systems “learn” from the examples they’re fed, if they don’t get enough examples of, say, black women during the learning stage, they’ll have a harder time recognizing them when deployed.
Similarly, the authors of the self-driving car study note that a couple of factors are likely fueling the disparity in their case. First, the object-detection models had mostly been trained on examples of light-skinned pedestrians. Second, the models didn’t place enough weight on learning from the few examples of dark-skinned people that they did have.
More heavily weighting that sample in the training data can help correct the bias, the researchers found. So can including more dark-skinned examples in the first place.
As for the broader problem of algorithmic bias, there are a couple of commonly proposed solutions. One is to make sure teams developing new technologies are racially diverse. If all team members are white, male, or both, it may not occur to them to check how their algorithm handles an image of a black woman. But if there’s a black woman in the room, it will probably occur to her, as MIT’s Joy Buolamwini has exemplified.
Another solution is to mandate that companies test their algorithms for bias and demonstrate that they meet certain fairness standards before they can be rolled out.
Kartik Hosanagar, the author of A Human’s Guide to Machine Intelligence, was not surprised when I told him the results of the self-driving car study, noting that “there have been so many stories” like this. Looking toward future solutions, he said, “I think an explicit test for bias is a more useful thing to do. To mandate that every team needs to have enough diversity is going to be hard because diversity can be many things: race, gender, nationality. But to say there are certain key things a company has to do — you have to test for race bias — I think that’s going to be more effective.”
These fixes aren’t mutually exclusive. And arguably, it’s in companies’ best interest to do everything they can to root out racial bias, before people of color are forced to take the brunt of it, literally.
source
https://www.vox.com/future-perfect/2019/3/5/1...-dark-skin

