Revolutionizing Medical Diagnostics with Advanced AI Defense
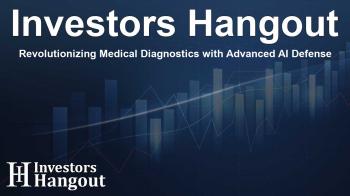
Innovative Defense System for Medical Digital Twins
This method enables medical digital twins to achieve 98% accuracy in breast cancer prediction, even under adversarial attacks.
Medical digital twins are sophisticated virtual models that mirror real biological systems, offering healthcare professionals a powerful tool for predicting and diagnosing diseases. However, these digital counterparts face significant challenges, particularly concerning cyberattacks that can distort data and lead to inaccurate medical assessments. To tackle this pressing issue, a team of researchers at Dongguk University has introduced a groundbreaking defense system known as Wavelet-Based Adversarial Training (WBAD).
Understanding the Importance of Medical Digital Twins
A digital twin serves as an exact simulation of a physical entity. By utilizing real-time data, these models can effectively test, simulate, and enhance various medical processes. In the field of healthcare, medical digital twins can generate detailed representations of biological systems, thus predicting diseases and evaluating the efficacy of medical treatments.
Nevertheless, the integrity of these systems can be compromised by adversarial attacks. These attacks can subtly alter input data, leading to erroneous predictions, potentially endangering patient safety. As such, ensuring the security of medical digital twins is crucial in maintaining their reliability and effectiveness.
Wavelet-Based Adversarial Training: A Novel Approach
The research team from Dongguk University, alongside experts from Oregon State University, spearheaded by Professor Insoo Sohn, has crafted a state-of-the-art algorithm designed to protect medical digital twins from cyber threats. The proposed Wavelet-Based Adversarial Training (WBAD) represents a pioneering advancement in digital twin security.
According to Professor Sohn, "This study marks the first initiative within Digital Twin Security, showcasing a secure medical digital twin system that features a unique two-stage defense against cyberattacks. This multifaceted strategy incorporates wavelet denoising and adversarial training to bolster system resilience.”
Testing the Defense System
The team applied their defense mechanism to a digital twin specifically designed for breast cancer diagnosis through thermography images. This imaging technique captures temperature variations within the body; tumors often manifest as elevated temperature areas due to increased blood circulation and metabolic activity. Using the Discrete Wavelet Transform, the researchers extracted essential insights from these images to produce Initial Feature Point Images.
The initial model achieved an accuracy rate of 92% for breast cancer predictions. However, the model's performance markedly declined to 5% when exposed to various adversarial attacks, exposing vulnerabilities that needed to be addressed. To enhance the model's robustness, the researchers implemented a two-layer defense mechanism. The initial level of protection involves wavelet denoising during the image preprocessing phase, which targets high-frequency noise that can mislead the algorithm. By applying soft thresholding, this technique successfully eliminates disruptive noise while maintaining crucial low-frequency features.
Enhancing Model Resilience
To further strengthen the model's defenses, the researchers incorporated an adversarial training phase. This step trains the machine learning model to identify and withstand adversarial manipulations. This dual-layered defense approach proved to be exceptionally effective, resulting in the model scoring an impressive 98% accuracy against certain attacks, such as the Fast Gradient Sign Method (FGSM), 93% against Projected Gradient Descent (PGD), and 90% against Carlini & Wagner (C&W) attacks.
Professor Sohn underscores the impact of their findings, stating “Our results reflect a transformative step forward in the realm of medical digital twin security, delivering a comprehensive and efficient defense against cyber threats while enhancing system performance and reliability.”
Concluding Thoughts on Medical Technology Security
The implications of this research extend far beyond the confines of academia, offering substantial promise for real-world applications in healthcare technology. As medical digital twins continue to evolve and integrate more deeply into diagnostic practices, ensuring their safety from malicious attacks becomes paramount. With innovative models like WBAD, the future of medical diagnostics looks brighter and significantly more secure.
Frequently Asked Questions
What are medical digital twins?
Medical digital twins are virtual models of human biological systems that mimic real-life processes to aid in disease prediction and treatment evaluation.
How does the Wavelet-Based Adversarial Training work?
WBAD employs a two-stage defense system that includes wavelet denoising to eliminate noise from images and adversarial training to enhance model resilience against data manipulation.
What were the initial accuracy rates of the breast cancer diagnostic model?
The model initially achieved a 92% accuracy rate in predicting breast cancer before being subjected to adversarial attacks.
What are adversarial attacks?
Adversarial attacks are targeted manipulations of input data designed to mislead models like digital twins, potentially resulting in incorrect predictions.
Who conducted this research?
The research was conducted by a team from Dongguk University, Republic of Korea, and Oregon State University, led by Professor Insoo Sohn.
About The Author
Contact Evelyn Baker privately here. Or send an email with ATTN: Evelyn Baker as the subject to contact@investorshangout.com.
About Investors Hangout
Investors Hangout is a leading online stock forum for financial discussion and learning, offering a wide range of free tools and resources. It draws in traders of all levels, who exchange market knowledge, investigate trading tactics, and keep an eye on industry developments in real time. Featuring financial articles, stock message boards, quotes, charts, company profiles, and live news updates. Through cooperative learning and a wealth of informational resources, it helps users from novices creating their first portfolios to experts honing their techniques. Join Investors Hangout today: https://investorshangout.com/
The content of this article is based on factual, publicly available information and does not represent legal, financial, or investment advice. Investors Hangout does not offer financial advice, and the author is not a licensed financial advisor. Consult a qualified advisor before making any financial or investment decisions based on this article. This article should not be considered advice to purchase, sell, or hold any securities or other investments. If any of the material provided here is inaccurate, please contact us for corrections.