(Total Views: 107)
Posted On: 03/13/2025 4:41:14 PM
Post# of 169
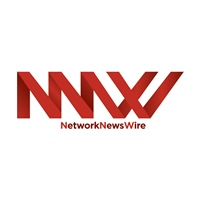
Why it is Crucial to Prepare Healthcare Data for Use in AI Models
The rate at which AI is being integrated in healthcare is accelerating. However, data quality is often lacking, and this reduces the effectiveness of many AI solutions tailored to the healthcare industry. Given that healthcare data is often siloed, the vast majority of this data is unstructured. This can pose challenges in the use of this data for AI models since the insights extracted could be biased or have other defects.
Data needs to be consistent in the way it is presented, its quality must be high and the formats need to be standardized. Otherwise, the data could be misinterpreted or the AI model can miss potentially useful insights.
Other challenges with medical data that ought to be addressed before the data is used by AI models include inaccuracies in the data, lack of validity and incomplete data. These issues of data quality can result in the model being misled to the extent that the AI perceives patterns which don’t exist in reality. Addressing these challenges before this data is deployed for use by an AI model is therefore vital.
The biggest hurdle in data preparation, especially healthcare data, lies in the unstructured or semi-structured nature of that data. A key step to fixing this defect is to get that raw information and transform it so that it matches an existing industry standard. For example, you could take a clinical terminology tool and use it to codify the available clinical notes so that this data aligns with an existing industry standard.
There are six core attributes which one must ensure when preparing their data for use by an AI system. These are timeliness, consistency, completeness, data integrity, validity and accuracy. Healthcare entities that keep an eye on these elements have a high chance of minimizing errors when this data is used by an AI system and the use of that data will be efficient.
Data governance is key to ensuring that all the data used is good and appropriate. For example, the data governance system has to have mechanisms through which lab data can be verified to confirm that it conforms to an existing industry standard. This can prevent wrong codes finding their way into the data being relied upon to train an AI model or seek actionable insights. The data governance system should also have a mechanism to incorporate all the coding system updates issued from time to time so that the data remains classified in a way that stays relevant as codes change or are updated. This plugs all gaps and eliminates bias in the insights obtained.
Companies like HealthLynked Corp. (OTCQB: HLYK) that specialize in offering digital healthcare data solutions may have a lot of lessons that other healthcare-linked organizations can learn from in order to make their data AI-ready.
NOTE TO INVESTORS: The latest news and updates relating to HealthLynked Corp. (OTCQB: HLYK) are available in the company’s newsroom at https://ibn.fm/HLYK
Please see full terms of use and disclaimers on the BioMedWire website applicable to all content provided by BMW, wherever published or re-published: http://BMW.fm/Disclaimer
The rate at which AI is being integrated in healthcare is accelerating. However, data quality is often lacking, and this reduces the effectiveness of many AI solutions tailored to the healthcare industry. Given that healthcare data is often siloed, the vast majority of this data is unstructured. This can pose challenges in the use of this data for AI models since the insights extracted could be biased or have other defects.
Data needs to be consistent in the way it is presented, its quality must be high and the formats need to be standardized. Otherwise, the data could be misinterpreted or the AI model can miss potentially useful insights.
Other challenges with medical data that ought to be addressed before the data is used by AI models include inaccuracies in the data, lack of validity and incomplete data. These issues of data quality can result in the model being misled to the extent that the AI perceives patterns which don’t exist in reality. Addressing these challenges before this data is deployed for use by an AI model is therefore vital.
The biggest hurdle in data preparation, especially healthcare data, lies in the unstructured or semi-structured nature of that data. A key step to fixing this defect is to get that raw information and transform it so that it matches an existing industry standard. For example, you could take a clinical terminology tool and use it to codify the available clinical notes so that this data aligns with an existing industry standard.
There are six core attributes which one must ensure when preparing their data for use by an AI system. These are timeliness, consistency, completeness, data integrity, validity and accuracy. Healthcare entities that keep an eye on these elements have a high chance of minimizing errors when this data is used by an AI system and the use of that data will be efficient.
Data governance is key to ensuring that all the data used is good and appropriate. For example, the data governance system has to have mechanisms through which lab data can be verified to confirm that it conforms to an existing industry standard. This can prevent wrong codes finding their way into the data being relied upon to train an AI model or seek actionable insights. The data governance system should also have a mechanism to incorporate all the coding system updates issued from time to time so that the data remains classified in a way that stays relevant as codes change or are updated. This plugs all gaps and eliminates bias in the insights obtained.
Companies like HealthLynked Corp. (OTCQB: HLYK) that specialize in offering digital healthcare data solutions may have a lot of lessons that other healthcare-linked organizations can learn from in order to make their data AI-ready.
NOTE TO INVESTORS: The latest news and updates relating to HealthLynked Corp. (OTCQB: HLYK) are available in the company’s newsroom at https://ibn.fm/HLYK
Please see full terms of use and disclaimers on the BioMedWire website applicable to all content provided by BMW, wherever published or re-published: http://BMW.fm/Disclaimer

