MicroCloud Hologram Enhances Anomaly Detection with DeepSeek
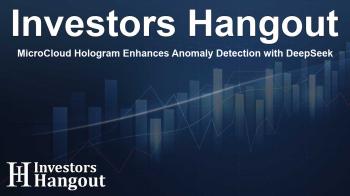
MicroCloud Hologram Inc. Optimizes Anomaly Detection
Recently, MicroCloud Hologram Inc. (NASDAQ: HOLO) made a significant advancement in the realm of technology services by deep optimizing stacked sparse autoencoders through its DeepSeek open-source model. This innovative step injects new life into anomaly detection technology, presenting a highly effective solution for various applications.
The Importance of Data Quality
Data quality serves as a cornerstone for the performance of any model. During the data preprocessing stage, the behavioral data often encompasses various features with differing dimensions and numerical ranges. To mitigate dimensional disparities and enhance model training effectiveness, HOLO implements a normalization processing method.
Understanding Normalization
Normalization is a widely employed data preprocessing technique that scales data within a specified range, commonly between 0 to 1 or -1 to 1. This approach allows for more equitable comparison and analysis of data from differing features, ensuring that no specific feature overly influences the model training due to its larger scale. In HOLO's anomaly detection project, normalization not only boosts model training efficiency but also establishes a solid foundation for effective feature extraction.
Advanced Model Architecture
Once data preprocessing is completed, the next logical step is feeding this refined data into the stacked sparse autoencoder model. This deep learning architecture consists of multiple autoencoder layers, each engineered to extract features at distinct levels. HOLO harnesses the power of the DeepSeek model to dynamically modulate the sparsity constraints, ensuring that the features extracted by each layer are both sparse and representative.
Sparsity Constraints for Better Learning
An autoencoder is fundamentally an unsupervised learning model designed to encode input data into a lower-dimensional representation through its encoder, subsequently reconstructing the original input as accurately as possible via its decoder. By adjusting the sparsity constraints, the model can more effectively capture critical information, reducing redundancy and enhancing overall data representation.
Innovative Training Techniques
Through the utilization of the DeepSeek model, HOLO has redefined the optimization process of stacked sparse autoencoders. This model adopts a layered training strategy that is both greedy and methodical, optimizing each autoencoder layer's parameters incrementally. The strategy begins with training lower layers to grasp the fundamental features of input data, and then taking the output from these layers as input for the next, allowing for a deepening extraction of complex data relationships.
Building Robust Features
HOLO's approach also integrates noise addition to the input data during training. This denoising method compels the model to reconstruct the original data amidst interference, honing its ability to identify anomalies even when faced with noisy real-world conditions. Random noise is systematically introduced, training the model to derive more resilient feature representations, thus ensuring high accuracy under diverse scenarios.
Regularization Techniques in Training
Along with the denoising approach, HOLO employs Dropout as a regularization technique to diminish the risk of overfitting. In deep learning, overfitting can result in models that excel on training data but falter on unseen samples. To counter this, HOLO intentionally drops a subset of neurons during the training for the stacked sparse autoencoder. By doing this, the model becomes less dependent on specific neurons, fostering the development of more general and robust feature representations.
Efficiency Through Distributed Computing
The DeepSeek model also employs a distributed computing framework to allocate training tasks across multiple nodes, significantly improving training speed and efficiency. Initial pretraining on the stacked sparse autoencoder allows the model to learn general feature representations swiftly, which can then be fine-tuned for optimal performance. This strategic combination accelerates model convergence, leading to enhanced results that are beneficial to various industry applications.
About MicroCloud Hologram Inc.
MicroCloud Hologram Inc. is committed to delivering premier holographic technology services globally. Their offerings encompass high-precision holographic LiDAR solutions, innovative technical holographic imaging solutions, and comprehensive design for holographic LiDAR sensor chips. Additionally, they focus on providing reliable advanced driver assistance systems (ADAS) through intelligent vision technology and their proprietary holographic digital twin resources. These resources capture real-world objects in three-dimensional holographic formats, enriching various sectors and applications.
Frequently Asked Questions
What is the significance of normalization in data processing?
Normalization ensures that various features are comparable, improving model training efficiency by eliminating scale biases.
How does the DeepSeek model improve model training?
The DeepSeek model optimizes the training process through dynamic adjustments of sparsity constraints, enhancing feature extraction.
What is the purpose of adding noise during training?
Addition of noise during training encourages the model to learn robust feature representations for accurate anomaly detection.
What role does Dropout play in model training?
Dropout prevents overfitting by randomly omitting neurons during training, promoting robust learning of general features.
What innovative technologies does MicroCloud offer?
MicroCloud provides leading holographic technology services, including high-precision LiDAR solutions and holographic digital twins, enhancing various applications.
About The Author
Contact Owen Jenkins privately here. Or send an email with ATTN: Owen Jenkins as the subject to contact@investorshangout.com.
About Investors Hangout
Investors Hangout is a leading online stock forum for financial discussion and learning, offering a wide range of free tools and resources. It draws in traders of all levels, who exchange market knowledge, investigate trading tactics, and keep an eye on industry developments in real time. Featuring financial articles, stock message boards, quotes, charts, company profiles, and live news updates. Through cooperative learning and a wealth of informational resources, it helps users from novices creating their first portfolios to experts honing their techniques. Join Investors Hangout today: https://investorshangout.com/
The content of this article is based on factual, publicly available information and does not represent legal, financial, or investment advice. Investors Hangout does not offer financial advice, and the author is not a licensed financial advisor. Consult a qualified advisor before making any financial or investment decisions based on this article. This article should not be considered advice to purchase, sell, or hold any securities or other investments. If any of the material provided here is inaccurate, please contact us for corrections.