Innovative Transistor Design Paving the Way for AI Advancements
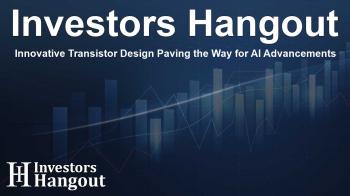
Transforming AI with New Transistor Technology
In a groundbreaking development in the field of artificial intelligence, researchers from the National University of Singapore have made a significant advancement in neuromorphic computing. Led by Associate Professor Mario Lanza, their team has brilliantly engineered a new computing cell that can replicate the functions of electronic neurons and synapses using a conventional silicon transistor. This revolutionary work is set to change how we view computing and AI.
The Concepts Behind Electronic Neurons and Synapses
Electronic neurons and synapses are crucial components of next-generation artificial neural networks. Unlike traditional computing systems that separate processing and memory, these innovative systems process and store data simultaneously within the same unit. This unique architecture eliminates inefficient data transfer, resulting in faster and more energy-efficient computation.
Challenges with Traditional Transistor Designs
One of the main challenges with creating electronic neurons and synapses has been the large number of silicon transistors required for implementation. Typically, constructing a single electronic neuron necessitates around 18 transistors, while synapses may require six. The complexity of having multiple components increases size and costs considerably, presenting a significant barrier to widespread adoption.
A Breakthrough Using Conventional Technology
The innovative approach by Professor Lanza’s team involves a clever manipulation of a standard silicon transistor to emulate the functions of both neurons and synapses. By adjusting the resistance of the bulk terminal, they produce a physical effect known as "impact ionization," which creates a current spike akin to neuron activation. With additional resistance settings, the transistor can store charge resembling synaptic behavior, leading to significant efficiency gains.
Revolutionizing Computational Efficiency
This novel discovery dramatically reduces the required number of transistors for electronic neurons and synapses. Notably, it decreases electronic neurons' size by a factor of 18 and synapses by a factor of 6. Given the millions of neurons and synapses in contemporary artificial neural networks, such advancements represent a monumental leap in computational capabilities while greatly improving energy efficiency.
The Versatility of Neuro-Synaptic Memory
The team has also designed a two-transistor system known as Neuro-Synaptic Random Access Memory (NSRAM). This innovative memory type allows the transistor to switch between neuron and synapse modes effortlessly, demonstrating exceptional flexibility. Such versatility in manufacturing is crucial as it simplifies production while maintaining functional diversity.
Utilizing Traditional Transistor Technology
The transistors employed in these developments are not new-age models sourced from high-tech regions like Taiwan or Korea. Instead, they utilize conventional 180-nanometer node transistors that can be readily produced. Professor Lanza emphasizes the importance of recognizing that once the operational mechanisms are understood, advancements can be made through refined microelectronic designs.
A Shift in the AI Landscape
Dr. Sebastián Pazos, the first author of the research, highlighted a paradigm shift in semiconductors and artificial intelligence. Rather than focusing solely on reducing the size of transistors through brute force methods, the team’s work promotes an efficient model emphasizing neuronal and synaptic approaches. This new framework aims to democratize nanoelectronics, allowing wider contributions to advanced computing progress without the barrier of high-tech fabrication access.
Frequently Asked Questions
What is the main breakthrough in this transistor technology?
The main breakthrough is the ability to replicate electronic neuron and synapse functions using a single conventional silicon transistor, greatly reducing the number of components needed.
How does this new technology impact traditional computing?
This technology offers faster and more energy-efficient computing by allowing simultaneous data processing and storage, unlike traditional systems that separate these functions.
What is Neuro-Synaptic Random Access Memory (NSRAM)?
NSRAM is a two-transistor system designed for flexibility, enabling the switching between neuron and synapse modes, which enhances manufacturing efficiency.
Can this technology be produced using existing methods?
Yes, the transistors utilized are traditional 180-nanometer node transistors that can be produced by local companies, making them accessible for wider application.
What future implications does this technology have for AI development?
This development could lead to significantly improved artificial neural networks, allowing them to handle more complex tasks with lower energy consumption, effectively enhancing overall AI capabilities.
About The Author
Contact Caleb Price privately here. Or send an email with ATTN: Caleb Price as the subject to contact@investorshangout.com.
About Investors Hangout
Investors Hangout is a leading online stock forum for financial discussion and learning, offering a wide range of free tools and resources. It draws in traders of all levels, who exchange market knowledge, investigate trading tactics, and keep an eye on industry developments in real time. Featuring financial articles, stock message boards, quotes, charts, company profiles, and live news updates. Through cooperative learning and a wealth of informational resources, it helps users from novices creating their first portfolios to experts honing their techniques. Join Investors Hangout today: https://investorshangout.com/
The content of this article is based on factual, publicly available information and does not represent legal, financial, or investment advice. Investors Hangout does not offer financial advice, and the author is not a licensed financial advisor. Consult a qualified advisor before making any financial or investment decisions based on this article. This article should not be considered advice to purchase, sell, or hold any securities or other investments. If any of the material provided here is inaccurate, please contact us for corrections.