Innovative Quantum Tech Revolutionizes Machine Learning Strategies
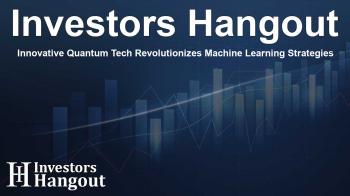
Revolutionizing Machine Learning with Quantum Technology
MicroAlgo Inc. (NASDAQ: MLGO) has recently made significant strides in machine learning by developing a new classifier auto-optimization technology grounded in Variational Quantum Algorithms (VQA). This groundbreaking approach addresses the challenges faced by traditional quantum classifiers by dramatically simplifying parameter updates during training. The result is a marked improvement in computational efficiency, setting a new standard in the practical application of quantum machine learning.
Challenges of Traditional Quantum Classifiers
Although quantum classifiers possess the theoretical capacity to enhance machine learning tasks, they are not without their challenges. Conventional quantum classifiers are plagued by the need for deep quantum circuits to effectively map features. As data volume grows, the complexity involved in updating parameters increases, leading to extended training times. This accumulation of complexities poses questions about the practicality of these models in real-world scenarios.
MicroAlgo's Innovative Solutions
MicroAlgo's innovative classifier optimization technology simplifies this complexity through a dual focus: enhancing circuit design and optimizing algorithms. By adopting a streamlined quantum circuit structure, MicroAlgo minimizes the number of quantum gates, which, in turn, conserves computational resources. Furthermore, their cutting-edge parameter update strategies enable swift adjustments to parameters, thus accelerating the speed of training processes.
Parameter Optimization: A Critical Step
Parameter optimization within the training stages of VQA classifiers is essential. Standard VQA classifiers often depend on Parameterized Quantum Circuits (PQC), where adjusting each parameter involves intricate gradient computations. As circuits delve deeper, the parameter space becomes exponentially more complex, making convergence increasingly challenging. Noise and uncertainty in quantum measurements can add further instability to this process, complicating the model's optimization journey.
Advancements in Quantum Circuit Design
To tackle these challenges, MicroAlgo implements various methods to streamline the design and operation of its quantum circuits. For instance, the Adaptive Circuit Pruning (ACP) technique dynamically alters circuit structures to eliminate unnecessary parameters while preserving effectiveness. This significant reduction in the number of parameters not only cuts down computational costs but also optimizes overall performance.
Hamiltonian Transformation Optimization
Additionally, MicroAlgo has introduced Hamiltonian Transformation Optimization (HTO). This innovative method modifies the Hamiltonian representation of the quantum circuit, shortening the search paths within the parameter space. Experiments indicate that this optimization can decrease computational complexity drastically, with results showing improvements by a factor of ten, all while ensuring classification accuracy remains intact.
Regularization Strategies to Combat Overfitting
To further improve model performance, MicroAlgo applies a novel Quantum Entanglement Regularization (QER) strategy. This technique dynamically modulates quantum entanglement strength during training, effectively curtailing overfitting tendencies. By adopting an energy landscape adjustment strategy, the model aids optimization algorithms in quickly identifying the global optimum and lessening local optima's impact.
Ensuring Robustness Against Noise
One of the pressing concerns with present quantum devices is noise interference. MicroAlgo addresses this through Variational Quantum Error Correction (VQEC). This approach not only enhances the model's robustness but also actively learns and compensates for errors that arise during training, leading to improved stability in noisy environments.
The remarkable advancements brought forth by MicroAlgo in classifier auto-optimization contribute to a revolutionary shift in quantum machine learning. By decisively reducing the computational complexity of parameter updates and embracing advanced regularization techniques, they enhance both training speed and model generalization. These developments represent substantial achievements in theory while demonstrating enhanced performance in practical applications.
Future Prospects in Quantum Machine Learning
The ongoing evolution of quantum computing hardware fosters increasing applications for this technology. MicroAlgo's innovations will likely pave the way for broader real-world implementation of quantum intelligent computing, entering a new stage of practical usefulness. Their advancements, coupled with the synergies of quantum computing and AI, will undeniably mark critical milestones in technology's future.
About MicroAlgo Inc.
MicroAlgo Inc., is a Cayman Islands exempted company committed to developing and applying tailored central processing algorithms. Their services encompass a broad spectrum of algorithm optimizations that enhance customer performance without necessitating hardware upgrades. By combining software, hardware, and custom algorithms, MicroAlgo empowers its clients to expand their customer base, improve satisfaction, manage costs, and progress toward their technical objectives.
Frequently Asked Questions
What is the significance of MicroAlgo's technology?
MicroAlgo's technology enhances computational efficiency in quantum machine learning, making practical applications more viable.
How does this technology improve training speed?
The classifier auto-optimization technology streamlines parameter updates and overall circuit design, significantly reducing training time.
What challenges do traditional quantum classifiers face?
Challenges include high computational complexity and prolonged training times due to the need for deep quantum circuits.
What are the unique features of MicroAlgo's approach?
Innovative methods like Adaptive Circuit Pruning and Hamiltonian Transformation Optimization set MicroAlgo apart, optimizing performance while reducing costs.
How does noise affect quantum machine learning?
Noise can disrupt training and performance; MicroAlgo's noise correction strategies enhance model stability and reliability in real-world applications.
About The Author
Contact Thomas Cooper privately here. Or send an email with ATTN: Thomas Cooper as the subject to contact@investorshangout.com.
About Investors Hangout
Investors Hangout is a leading online stock forum for financial discussion and learning, offering a wide range of free tools and resources. It draws in traders of all levels, who exchange market knowledge, investigate trading tactics, and keep an eye on industry developments in real time. Featuring financial articles, stock message boards, quotes, charts, company profiles, and live news updates. Through cooperative learning and a wealth of informational resources, it helps users from novices creating their first portfolios to experts honing their techniques. Join Investors Hangout today: https://investorshangout.com/
The content of this article is based on factual, publicly available information and does not represent legal, financial, or investment advice. Investors Hangout does not offer financial advice, and the author is not a licensed financial advisor. Consult a qualified advisor before making any financial or investment decisions based on this article. This article should not be considered advice to purchase, sell, or hold any securities or other investments. If any of the material provided here is inaccurate, please contact us for corrections.